人工智能在慢加急性肝衰竭预后预测模型中的研究现状
DOI: 10.12449/JCH240927
Current status of research on artificial intelligence in prognostic prediction models for acute-on-chronic liver failure
-
摘要: 慢加急性肝衰竭(ACLF)是在慢性肝病基础上出现的急性肝功能恶化,且以肝脏和/或肝外器官衰竭和短期高病死率为主要特征的复杂临床综合征。目前缺乏有效的治疗手段,内科综合治疗下病死率高达50%~90%。开发简单快捷、准确性高的ACLF预后预测模型,能帮助临床医师早期准确判断ACLF患者预后,识别预后不良患者,从而实施早期干预,可在一定程度上改善预后,有助于降低病死率。随着计算机科学的不断发展,数据处理能力愈发强大,人工智能越来越受到重视,在肝脏疾病的诊断、治疗、预后预测等多方面均有应用。本文结合国内外研究现状,对常见的ACLF预后模型和机器学习预后预测模型进行综述,总结最新研究进展,为ACLF预后预测模型未来发展提供新思路。Abstract: Acute-on-chronic liver failure (ACLF) is a complex clinical syndrome of acute liver function deterioration on the basis of chronic liver diseases, characterized by hepatic and/or extra-hepatic organ failure and a high short-term mortality rate. At present, there is still a lack of effective treatment methods, and the mortality rate of ACLF reaches 50% — 90% after comprehensive medical treatment. A simple, rapid, and accurate prognostic prediction model for ACLF can help clinicians accurately judge the prognosis of ACLF patients in the early stage, identify the patients with poor prognosis, and provide early interventions, which can improve patient prognosis to some extent and help to reduce mortality rates. With the continuous development of computer science and increasingly powerful data processing capabilities, artificial intelligence is gaining more attention and has been applied in various aspects of liver diseases including diagnosis, treatment, and prognostic prediction. With reference to the current status of research in China and globally, this article reviews the common prognostic models for ACLF and machine learning-based prognostic prediction models and summarizes the latest research advances, in order to provide new perspectives for the future development of prognostic prediction models for ACLF.
-
Key words:
- Acute-On-Chronic Liver Failure /
- Artificial Intelligence /
- Machine Learning /
- Prognosis
-
表 1 传统ACLF预后模型总结及比较
Table 1. The summary and comparison of common prognostic models for ACLF patients
评分模型 构建模型样本量 纳入指标 模型构建方式 优点 缺点 CTP[9] - HE分级、腹腔积液程度、TBil、Alb、PT 分层分析 计算简单,数据易获取,多方面评估基础肝功能情况 未对患者一般情况及其他器官进行评估 MELD[10] - TBil、INR、Cr、病因、年龄 3.78×ln(TBil)+11.2×ln(INR)+ 9.57×ln(Cr)+6.43×(胆汁性或酒精性0,其他1)(线性回归) 纳入年龄和病因,评估患者的一般状况 未对感染、肝性脑病等预后高危因素进行评价 APACHEⅡ[11] 100例酒精相关性ACLF APS、CPS 线性回归 客观地反映出患者疾病严重程度 指标涉及面广泛,复杂且难以实施 CLIF-C[12] 1 349例CANONIC研究患者 PaO2/FiO2、SPO2/FiO2、WBC、HE分级、TBil、Cr、INR、MAP CLIF-C ACLF=10×[0.33×CLIF-OF+0.04×年龄+0.63×ln(WBC)-2](线性回归) 评估了除肝脏以外的循环系统、呼吸系统等器官的情况 计算繁琐,基于西方国家酒精性肝硬化患者,对其他病因患者预测性能未得到验证 AARC-ACLF[13] 1 402例符合APASL定义的ACLF患者 INR、Cr、TBil、HE分级、血乳酸 分层分析 计算简单,检验结果易获取,样本量大 缺乏大样本量验证模型,对其他标准下的预测性能不详 COSSH-ACLF[14] 1 322例重症乙型肝炎患者 INR、TBil、血尿素、年龄、中性粒细胞数量、HE分级 1.649×ln(INR)+0.457 8×HE评分+0.425×ln(中性粒胞)+ 0.396×ln(TBil)+0.576×ln(尿素氮)+0.33×年龄(线性回归) 基于大样本量HBV-ACLF,对HBV-ACLF患者预测价值较高 缺乏大样本量验证模型,对其他病因ACLF患者预测性能不详 注:APACHE Ⅱ,急性生理慢性健康评分;CLIF-C,慢性肝脏疾病研究协作组评分;AARC-ACLF,亚太肝病学会慢加急性肝衰竭评分;COSSH-ACLF,中国肝病协作组慢加急性肝功能衰竭评分;INR,国际标准化比值;PT,凝血酶原时间;Cr,血清肌酐;APS,急性生理评分;CPS,慢性健康评分;PaO2,动脉血氧浓度;FiO2,吸入氧浓度;SPO2,血氧饱和度;MAP,平均动脉压;APASL,亚太肝病学会;CLIF-OF,CLIF-器官衰竭评分系统。
表 2 ACLF中的机器学习模型
Table 2. Mechine learning model of ACLF
作者 方法 诊断标准 比较对象 28天预测准确性 90天预测准确性 Zheng等[23] ANN APASL-ACLF MELD - AUC=0.765,P<0.001 Hou等[24] ANN APASL-ACLF MELD、MELD-Na、CTP、
CLIF-ACLF
AUC=0.748,95%CI:0.673~0.822 AUC=0.754,95%CI:0.697~0.812 Shi等[25] CART、LR APASL-CLIF MELD - CART:AUC=0.896,LR:AUC=0.914,P值均<0.001 Verma等[26] XGB-CV APASL-ACLF XGB、GBM、RF、DL、NB、
FLM、DT、SVM、MELD
AUC=0.878,95%CI:0.854~0.898(30天) XGB-CV:AUC=0.919,95%CI:0.908~0.930 注:SVM,支持向量机。
-
[1] MOREAU R, JALAN R, GINES P, et al. Acute-on-chronic liver failure is a distinct syndrome that develops in patients with acute decompensation of cirrhosis[J]. Gastroenterology, 2013, 144( 7): 1426- 1437. e 9. DOI: 10.1053/j.gastro.2013.02.042. [2] MIAO N, WANG FZ, ZHENG H, et al. Estimation of incidence of viral hepatitis B and analysis on case characteristics in China, 2013-2020[J]. Chin J Epidemiol, 2021, 42( 9): 1527- 1531. DOI: 10.3760/cma.j.cn112338-20210319-00227.缪宁, 王富珍, 郑徽, 等. 中国2013—2020年乙型肝炎发病情况估算和病例特征分析[J]. 中华流行病学杂志, 2021, 42( 9): 1527- 1531. DOI: 10.3760/cma.j.cn112338-20210319-00227. [3] ARROYO V, MOREAU R, JALAN R. Acute-on-chronic liver failure[J]. N Engl J Med, 2020, 382( 22): 2137- 2145. DOI: 10.1056/nejmra1914900. [4] JALAN R, GUSTOT T, FERNANDEZ J, et al.‘Equity’ and‘Justice’ for patients with acute-on chronic liver failure: A call to action[J]. J Hepatol, 2021, 75( 5): 1228- 1235. DOI: 10.1016/j.jhep.2021.06.017. [5] LING SB, JIANG GJ, QUE QY, et al. Liver transplantation in patients with liver failure: Twenty years of experience from China[J]. Liver Int, 2022, 42( 9): 2110- 2116. DOI: 10.1111/liv.15288. [6] LI P, LIANG X, LUO JJ, et al. Predicting the survival benefit of liver transplantation in HBV-related acute-on-chronic liver failure: An observational cohort study[J]. Lancet Reg Health West Pac, 2022, 32: 100638. DOI: 10.1016/j.lanwpc.2022.100638. [7] YANG LS, SHAN LL, SAXENA A, et al. Liver transplantation: A systematic review of long-term quality of life[J]. Liver Int, 2014, 34( 9): 1298- 1313. DOI: 10.1111/liv.12553. [8] HERNAEZ R, LIU Y, KRAMER JR, et al. Model for end-stage liver disease-sodium underestimates 90-day mortality risk in patients with acute-on-chronic liver failure[J]. J Hepatol, 2020, 73( 6): 1425- 1433. DOI: 10.1016/j.jhep.2020.06.005. [9] PUGH RN, MURRAY-LYON IM, DAWSON JL, et al. Transection of the oesophagus for bleeding oesophageal varices[J]. Br J Surg, 1973, 60( 8): 646- 649. DOI: 10.1002/bjs.1800600817. [10] MALINCHOC M, KAMATH PS, GORDON FD, et al. A model to predict poor survival in patients undergoing transjugular intrahepatic portosystemic shunts[J]. Hepatology, 2000, 31( 4): 864- 871. DOI: 10.1053/he.2000.5852. [11] KNAUS WA, ZIMMERMAN JE, WAGNER DP, et al. APACHE-acute physiology and chronic health evaluation: A physiologically based classification system[J]. Crit Care Med, 1981, 9( 8): 591- 597. DOI: 10.1097/00003246-198108000-00008. [12] HERNAEZ R, SOLÀ E, MOREAU R, et al. Acute-on-chronic liver failure: An update[J]. Gut, 2017, 66( 3): 541- 553. DOI: 10.1136/gutjnl-2016-312670. [13] CHOUDHURY A, JINDAL A, MAIWALL R, et al. Liver failure determines the outcome in patients of acute-on-chronic liver failure(ACLF): Comparison of APASL ACLF research consortium(AARC) and CLIF-SOFA models[J]. Hepatol Int, 2017, 11( 5): 461- 471. DOI: 10.1007/s12072-017-9816-z. [14] WU T, LI J, SHAO L, et al. Development of diagnostic criteria and a prognostic score for hepatitis B virus-related acute-on-chronic liver failure[J]. Gut, 2018, 67( 12): 2181- 2191. DOI: 10.1136/gutjnl-2017-314641. [15] DEO RC. Machine learning in medicine[J]. Circulation, 2015, 132( 20): 1920- 1930. DOI: 10.1161/CIRCULATIONAHA.115.001593. [16] ZHOU J, HU B, FENG W, et al. An ensemble deep learning model for risk stratification of invasive lung adenocarcinoma using thin-slice CT[J]. NPJ Digit Med, 2023, 6( 1): 119. DOI: 10.1038/s41746-023-00866-z. [17] WALSH JA, ROZYCKI M, YI E, et al. Application of machine learning in the diagnosis of axial spondyloarthritis[J]. Curr Opin Rheumatol, 2019, 31( 4): 362- 367. DOI: 10.1097/BOR.0000000000000612. [18] VAMATHEVAN J, CLARK D, CZODROWSKI P, et al. Applications of machine learning in drug discovery and development[J]. Nat Rev Drug Discov, 2019, 18( 6): 463- 477. DOI: 10.1038/s41573-019-0024-5. [19] TIAN D, YAN HJ, HUANG H, et al. Machine learning-based prognostic model for patients after lung transplantation[J]. JAMA Netw Open, 2023, 6( 5): e2312022. DOI: 10.1001/jamanetworkopen.2023.12022. [20] AHN JC, CONNELL A, SIMONETTO DA, et al. Application of artificial intelligence for the diagnosis and treatment of liver diseases[J]. Hepatology, 2021, 73( 6): 2546- 2563. DOI: 10.1002/hep.31603. [21] KINOSHITA F, TAKENAKA T, YAMASHITA T, et al. Development of artificial intelligence prognostic model for surgically resected non-small cell lung cancer[J]. Sci Rep, 2023, 13( 1): 15683. DOI: 10.1038/s41598-023-42964-8. [22] SIDEY-GIBBONS JAM, SIDEY-GIBBONS CJ. Machine learning in medicine: A practical introduction[J]. BMC Med Res Methodol, 2019, 19( 1): 64. DOI: 10.1186/s12874-019-0681-4. [23] ZHENG MH, SHI KQ, LIN XF, et al. A model to predict 3-month mortality risk of acute-on-chronic hepatitis B liver failure using artificial neural network[J]. J Viral Hepat, 2013, 20( 4): 248- 255. DOI: 10.1111/j.1365-2893.2012.01647.x. [24] HOU YX, ZHANG QQ, GAO FY, et al. Artificial neural network-based models used for predicting 28- and 90-day mortality of patients with hepatitis B-associated acute-on-chronic liver failure[J]. BMC Gastroenterol, 2020, 20( 1): 75. DOI: 10.1186/s12876-020-01191-5. [25] SHI KQ, ZHOU YY, YAN HD, et al. Classification and regression tree analysis of acute-on-chronic hepatitis B liver failure: Seeing the forest for the trees[J]. J Viral Hepat, 2017, 24( 2): 132- 140. DOI: 10.1111/jvh.12617. [26] VERMA N, CHOUDHURY A, SINGH V, et al. APASL-ACLF Research Consortium-Artificial Intelligence(AARC-AI) model precisely predicts outcomes in acute-on-chronic liver failure patients[J]. Liver Int, 2023, 43( 2): 442- 451. DOI: 10.1111/liv.15361. [27] LUO W, PHUNG D, TRAN T, et al. Guidelines for developing and reporting machine learning predictive models in biomedical research: A multidisciplinary view[J]. J Med Internet Res, 2016, 18( 12): e323. DOI: 10.2196/jmir.5870. [28] YU KH, BEAM AL, KOHANE IS. Artificial intelligence in healthcare[J]. Nat Biomed Eng, 2018, 2( 10): 719- 731. DOI: 10.1038/s41551-018-0305-z. [29] CAO ZJ, LI FD, XIANG XG, et al. Circulating cell death biomarker: Good candidates of prognostic indicator for patients with hepatitis B virus related acute-on-chronic liver failure[J]. Sci Rep, 2015, 5: 14240. DOI: 10.1038/srep14240. [30] ARIZA X, GRAUPERA I, COLL M, et al. Neutrophil gelatinase-associated lipocalin is a biomarker of acute-on-chronic liver failure and prognosis in cirrhosis[J]. J Hepatol, 2016, 65( 1): 57- 65. DOI: 10.1016/j.jhep.2016.03.002. [31] JUANOLA A, GRAUPERA I, ELIA C, et al. Urinary L-FABP is a promising prognostic biomarker of ACLF and mortality in patients with decompensated cirrhosis[J]. J Hepatol, 2022, 76( 1): 107- 114. DOI: 10.1016/j.jhep.2021.08.031. [32] PENG H, ZHANG Q, LUO L, et al. A prognostic model of acute-on-chronic liver failure based on sarcopenia[J]. Hepatol Int, 2022, 16( 4): 964- 972. DOI: 10.1007/s12072-022-10363-2. [33] HE TC, FONG JN, MOORE LW, et al. An imageomics and multi-network based deep learning model for risk assessment of liver transplantation for hepatocellular cancer[J]. Comput Med Imaging Graph, 2021, 89: 101894. DOI: 10.1016/j.compmedimag.2021.101894. -
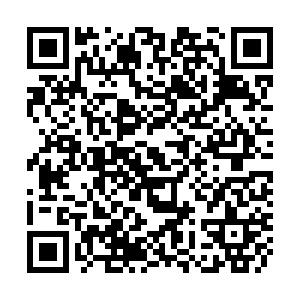
计量
- 文章访问数: 230
- HTML全文浏览量: 83
- PDF下载量: 39
- 被引次数: 0