人工智能在胰腺癌诊治中的应用现状
DOI: 10.12449/JCH241032
利益冲突声明:本文不存在任何利益冲突。
作者贡献声明:马昱负责设计论文框架,起草论文;贾峰负责关键点分析,论文修改;马昱、刘楷宇负责文献查找;刘亚辉负责拟定写作思路,指导撰写文章并最后定稿。
Current status of the application of artificial intelligence in the diagnosis and treatment of pancreatic cancer
-
摘要: 胰腺癌是消化系统常见的恶性肿瘤,早期诊断率低,手术病死率高,治愈率低,总体预后差。近年来,随着人工智能在医学领域的不断发展,机器学习、深度学习等人工智能技术被广泛应用于医学研究中。本文综述了近年来人工智能技术在胰腺癌筛查、诊断、治疗、并发症及预后预测等方面的应用,为人工智能在胰腺癌诊治中的应用提供依据和新思路。Abstract: Pancreatic cancer is a common malignant tumor of the digestive system, with a low early diagnosis rate, a high surgical mortality rate, a low cure rate, and a poor overall prognosis. In recent years, with the continuous development of artificial intelligence in the medical field, artificial intelligence techniques, such as machine learning and deep learning, have been widely used in medical research. This article reviews the application of artificial intelligence techniques in the screening, diagnosis, treatment, complications, and prognosis prediction of pancreatic cancer, so as to provide a basis and new ideas for the application of artificial intelligence in the diagnosis and treatment of pancreatic cancer.
-
Key words:
- Artificial Intelligence /
- Pancreatic Neoplasms /
- Machine Learning /
- Deep Learning
-
[1] KENNER B, CHARI ST, KELSEN D, et al. Artificial intelligence and early detection of pancreatic cancer: 2020 summative review[J]. Pancreas, 2021, 50( 3): 251- 279. DOI: 10.1097/MPA.0000000000001762. [2] KAUL V, ENSLIN S, GROSS SA. History of artificial intelligence in medicine[J]. Gastrointest Endosc, 2020, 92( 4): 807- 812. DOI: 10.1016/j.gie.2020.06.040. [3] CAI J, CHEN HD, LU M, et al. Advances in the epidemiology of pancreatic cancer: Trends, risk factors, screening, and prognosis[J]. Cancer Lett, 2021, 520: 1- 11. DOI: 10.1016/j.canlet.2021.06.027. [4] HUANG JJ, LOK V, NGAI CH, et al. Worldwide burden of, risk factors for, and trends in pancreatic cancer[J]. Gastroenterology, 2021, 160( 3): 744- 754. DOI: 10.1053/j.gastro.2020.10.007. [5] GRANATA V, FUSCO R, SETOLA SV, et al. Risk assessment and pancreatic cancer: Diagnostic management and artificial intelligence[J]. Cancers, 2023, 15( 2): 351. DOI: 10.3390/cancers15020351. [6] YANG JS, XU RY, WANG CC, et al. Early screening and diagnosis strategies of pancreatic cancer: A comprehensive review[J]. Cancer Commun, 2021, 41( 12): 1257- 1274. DOI: 10.1002/cac2.12204. [7] PEREIRA SP, OLDFIELD L, NEY A, et al. Early detection of pancreatic cancer[J]. Lancet Gastroenterol Hepatol, 2020, 5( 7): 698- 710. DOI: 10.1016/S2468-1253(19)30416-9. [8] STOFFEL EM, BRAND RE, GOGGINS M. Pancreatic cancer: Changing epidemiology and new approaches to risk assessment, early detection, and prevention[J]. Gastroenterology, 2023, 164( 5): 752- 765. DOI: 10.1053/j.gastro.2023.02.012. [9] BOURSI B, FINKELMAN B, GIANTONIO BJ, et al. A clinical prediction model to assess risk for pancreatic cancer among patients with new-onset diabetes[J]. Gastroenterology, 2017, 152( 4): 840- 850. DOI: 10.1053/j.gastro.2016.11.046. [10] PLACIDO D, YUAN B, HJALTELIN JX, et al. A deep learning algorithm to predict risk of pancreatic cancer from disease trajectories[J]. Nat Med, 2023, 29( 5): 1113- 1122. DOI: 10.1038/s41591-023-02332-5. [11] BLYUSS O, ZAIKIN A, CHEREPANOVA V, et al. Development of PancRISK, a urine biomarker-based risk score for stratified screening of pancreatic cancer patients[J]. Br J Cancer, 2020, 122( 5): 692- 696. DOI: 10.1038/s41416-019-0694-0. [12] CAO K, XIA YD, YAO JW, et al. Large-scale pancreatic cancer detection via non-contrast CT and deep learning[J]. Nat Med, 2023, 29( 12): 3033- 3043. DOI: 10.1038/s41591-023-02640-w. [13] Chinese Pancreatic Surgery Association, Chinese Society of Surgery, Chinese Medical Association. Guidelines for the diagnosis and treatment of pancreatic cancer in China(2021)[J]. Chin J Dig Surg, 2021, 20( 7): 713- 729. DOI: 10.3760/cma.j.cn115610-20210618-00289.中华医学会外科学分会胰腺外科学组. 中国胰腺癌诊治指南(2021)[J]. 中华消化外科杂志, 2021, 20( 7): 713- 729. DOI: 10.3760/cma.j.cn115610-20210618-00289. [14] MIZRAHI JD, SURANA R, VALLE JW, et al. Pancreatic cancer[J]. Lancet, 2020, 395( 10242): 2008- 2020. DOI: 10.1016/S0140-6736(20)30974-0. [15] CHEN PT, WU TH, WANG PC, et al. Pancreatic cancer detection on CT scans with deep learning: A nationwide population-based study[J]. Radiology, 2023, 306( 1): 172- 182. DOI: 10.1148/radiol.220152. [16] MA H, LIU ZX, ZHANG JJ, et al. Construction of a convolutional neural network classifier developed by computed tomography images for pancreatic cancer diagnosis[J]. World J Gastroenterol, 2020, 26( 34): 5156- 5168. DOI: 10.3748/wjg.v26.i34.5156. [17] MUKHERJEE S, PATRA A, KHASAWNEH H, et al. Radiomics-based machine-learning models can detect pancreatic cancer on prediagnostic computed tomography scans at a substantial lead time before clinical diagnosis[J]. Gastroenterology, 2022, 163( 5): 1435- 1446. DOI: 10.1053/j.gastro.2022.06.066. [18] MARYA NB, POWERS PD, CHARI ST, et al. Utilisation of artificial intelligence for the development of an EUS-convolutional neural network model trained to enhance the diagnosis of autoimmune pancreatitis[J]. Gut, 2021, 70( 7): 1335- 1344. DOI: 10.1136/gutjnl-2020-322821. [19] TONOZUKA R, ITOI T, NAGATA N, et al. Deep learning analysis for the detection of pancreatic cancer on endosonographic images: A pilot study[J]. J Hepatobiliary Pancreat Sci, 2021, 28( 1): 95- 104. DOI: 10.1002/jhbp.825. [20] HUANG BW, HUANG HR, ZHANG ST, et al. Artificial intelligence in pancreatic cancer[J]. Theranostics, 2022, 12( 16): 6931- 6954. DOI: 10.7150/thno.77949. [21] MAHMOUDI T, KOUZAHKANAN ZM, RADMARD AR, et al. Segmentation of pancreatic ductal adenocarcinoma(PDAC) and surrounding vessels in CT images using deep convolutional neural networks and texture descriptors[J]. Sci Rep, 2022, 12( 1): 3092. DOI: 10.1038/s41598-022-07111-9. [22] XIE TS, WANG XY, LI ML, et al. Pancreatic ductal adenocarcinoma: A radiomics nomogram outperforms clinical model and TNM staging for survival estimation after curative resection[J]. Eur Radiol, 2020, 30( 5): 2513- 2524. DOI: 10.1007/s00330-019-06600-2. [23] WITKIEWICZ AK, MCMILLAN EA, BALAJI U, et al. Whole-exome sequencing of pancreatic cancer defines genetic diversity and therapeutic targets[J]. Nat Commun, 2015, 6: 6744. DOI: 10.1038/ncomms7744. [24] BAGANTE F, SPOLVERATO G, RUZZENENTE A, et al. Artificial neural networks for multi-omics classifications of hepato-pancreato-biliary cancers: Towards the clinical application of genetic data[J]. Eur J Cancer, 2021, 148: 348- 358. DOI: 10.1016/j.ejca.2021.01.049. [25] WEI Q, RAMSEY SA. Predicting chemotherapy response using a variational autoencoder approach[J]. BMC Bioinformatics, 2021, 22( 1): 453. DOI: 10.1186/s12859-021-04339-6. [26] CHEN DS, MELLMAN I. Elements of cancer immunity and the cancer-immune set point[J]. Nature, 2017, 541( 7637): 321- 330. DOI: 10.1038/nature21349. [27] BIAN Y, LIU YF, LI J, et al. Machine learning for computed tomography radiomics: Prediction of tumor-infiltrating lymphocytes in patients with pancreatic ductal adenocarcinoma[J]. Pancreas, 2022, 51( 5): 549- 558. DOI: 10.1097/MPA.0000000000002069. [28] WATSON MD, BAIMAS-GEORGE MR, MURPHY KJ, et al. Pure and hybrid deep learning models can predict pathologic tumor response to neoadjuvant therapy in pancreatic adenocarcinoma: A pilot study[J]. Am Surg, 2021, 87( 12): 1901- 1909. DOI: 10.1177/0003134820982557. [29] Study Group of Pancreatic Surgery in Chinese Society of Surgery of Chinese Medical Association; Pancreatic Disease Committee of Chinese Research Hospital Association; Editorial Board of Chinese Journal of Surgery. A consensus statement on the diagnosis, treatment, and prevention of common complications after pancreatic surgery(2017)[J]. Chin J Surg, 2017, 55( 5): 328- 334. DOI: 10.3760/cma.j.issn.0529-5815.2017.05.003.中华医学会外科学分会胰腺外科学组, 中国研究型医院学会胰腺病专业委员会, 中华外科杂志编辑部. 胰腺术后外科常见并发症诊治及预防的专家共识(2017)[J]. 中华外科杂志, 2017, 55( 5): 328- 334. DOI: 10.3760/cma.j.issn.0529-5815.2017.05.003. [30] CALLERY MP, PRATT WB, KENT TS, et al. A prospectively validated clinical risk score accurately predicts pancreatic fistula after pancreatoduodenectomy[J]. J Am Coll Surg, 2013, 216( 1): 1- 14. DOI: 10.1016/j.jamcollsurg.2012.09.002. [31] SHEN ZY, CHEN HD, WANG WS, et al. Machine learning algorithms as early diagnostic tools for pancreatic fistula following pancreaticoduodenectomy and guide drain removal: A retrospective cohort study[J]. Int J Surg, 2022, 102: 106638. DOI: 10.1016/j.ijsu.2022.106638. [32] YOO J, YOON SH, LEE DH, et al. Body composition analysis using convolutional neural network in predicting postoperative pancreatic fistula and survival after pancreatoduodenectomy for pancreatic cancer[J]. Eur J Radiol, 2023, 169: 111182. DOI: 10.1016/j.ejrad.2023.111182. [33] KAMBAKAMBA P, MANNIL M, HERRERA PE, et al. The potential of machine learning to predict postoperative pancreatic fistula based on preoperative, non-contrast-enhanced CT: A proof-of-principle study[J]. Surgery, 2020, 167( 2): 448- 454. DOI: 10.1016/j.surg.2019.09.019. [34] HAN IW, CHO K, RYU Y, et al. Risk prediction platform for pancreatic fistula after pancreatoduodenectomy using artificial intelligence[J]. World J Gastroenterol, 2020, 26( 30): 4453- 4464. DOI: 10.3748/wjg.v26.i30.4453. [35] WALCZAK S, VELANOVICH V. An evaluation of artificial neural networks in predicting pancreatic cancer survival[J]. J Gastrointest Surg, 2017, 21( 10): 1606- 1612. DOI: 10.1007/s11605-017-3518-7. [36] LIN JX, YIN MY, LIU L, et al. The development of a prediction model based on random survival forest for the postoperative prognosis of pancreatic cancer: A SEER-based study[J]. Cancers, 2022, 14( 19): 4667. DOI: 10.3390/cancers14194667. [37] HE M, CHEN XY, WELS M, et al. Computed tomography-based radiomics evaluation of postoperative local recurrence of pancreatic ductal adenocarcinoma[J]. Acad Radiol, 2023, 30( 4): 680- 688. DOI: 10.1016/j.acra.2022.05.019. [38] YOKOYAMA S, HAMADA T, HIGASHI M, et al. Predicted prognosis of patients with pancreatic cancer by machine learning[J]. Clin Cancer Res, 2020, 26( 10): 2411- 2421. DOI: 10.1158/1078-0432.CCR-19-1247. [39] LEE W, PARK HJ, LEE HJ, et al. Preoperative data-based deep learning model for predicting postoperative survival in pancreatic cancer patients[J]. Int J Surg, 2022, 105: 106851. DOI: 10.1016/j.ijsu.2022.106851. [40] KUMAR V, GU YH, BASU S, et al. Radiomics: The process and the challenges[J]. Magn Reson Imaging, 2012, 30( 9): 1234- 1248. DOI: 10.1016/j.mri.2012.06.010. [41] VARGHESE BA, CEN SY, HWANG DH, et al. Texture analysis of imaging: What radiologists need to know[J]. AJR Am J Roentgenol, 2019, 212( 3): 520- 528. DOI: 10.2214/AJR.18.20624. [42] KATTA MR, KALLURU PKR, BAVISHI DA, et al. Artificial intelligence in pancreatic cancer: Diagnosis, limitations, and the future prospects-a narrative review[J]. J Cancer Res Clin Oncol, 2023, 149( 9): 6743- 6751. DOI: 10.1007/s00432-023-04625-1. [43] LIANG ZX, YE LS, YANG Y. Application of artificial intelligence in liver transplantation[J]. J Clin Hepatol, 2022, 38( 1): 30- 34. DOI: 10.3969/j.issn.1001-5256.2022.01.005.梁智星, 叶林森, 杨扬. 人工智能在肝移植中的应用[J]. 临床肝胆病杂志, 2022, 38( 1): 30- 34. DOI: 10.3969/j.issn.1001-5256.2022.01.005. -
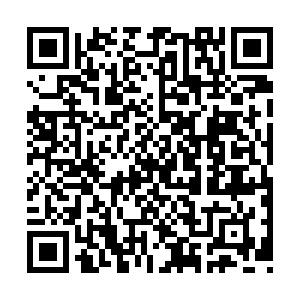
计量
- 文章访问数: 612
- HTML全文浏览量: 76
- PDF下载量: 17
- 被引次数: 0