人工智能在原发性肝癌诊疗中的应用
DOI: 10.3969/j.issn.1001-5256.2022.01.004
利益冲突声明:所有作者均声明不存在利益冲突。
作者贡献声明:方驰华负责选题,拟定写作思路和最终定稿;蔡伟负责撰写论文。
Application of artificial intelligence in the diagnosis and treatment of primary liver cancer
-
摘要: 在医疗大数据时代, 人工智能技术在医学中的应用日益广泛。通过对海量医学数据进行高效管理、信息挖掘后可以得到关于疾病发生发展、生存预后等有益信息。近年来, 人工智能技术在原发性肝癌中的应用亦取得了一些成果。本文将详述其在肝癌诊断和治疗中的应用现状及前景。Abstract: In the era of medical big data, artificial intelligence is increasingly widely used in medicine. Efficient management and information mining of massive medical data can obtain useful information on disease development, progression, survival, and prognosis. In recent years, some achievements have been made in the application of artificial intelligence in primary liver cancer. This article elaborates on the current status and prospects of its application in the diagnosis and treatment of liver cancer.
-
Key words:
- Liver Neoplasm /
- Artificial Intelligence /
- Convolutional Neural Networks
-
[1] JIMÉNEZ PÉREZ M, GRANDE RG. Application of artificial intelligence in the diagnosis and treatment of hepatocellular carcinoma: A review[J]. World J Gastroenterol, 2020, 26(37): 5617-5628. DOI: 10.3748/wjg.v26.i37.5617. [2] SIEGEL RL, MILLER KD, JEMAL A. Cancer statistics, 2020[J]. CA Cancer J Clin, 2020, 70(1): 7-30. DOI: 10.3322/caac.21590. [3] AN L, ZENG HM, ZHENG RS, et al. Liver cancer epidemiology in China, 2015[J]. Chin J Oncol, 2019, 41(10): 721-727. DOI: 10.3760/cma.j.issn.0253-3766.2019.10.001.安澜, 曾红梅, 郑荣寿, 等. 2015年中国肝癌流行情况分析[J]. 中华肿瘤杂志, 2019, 41(10): 721-727. DOI: 10.3760/cma.j.issn.0253-3766.2019.10.001. [4] BHARTI P, MITTAL D, ANANTHASIVAN R. Preliminary study of chronic liver classification on ultrasound images using an ensemble model[J]. Ultrason Imaging, 2018, 40(6): 357-379. DOI: 10.1177/0161734618787447. [5] LIU X, SONG JL, WANG SH, et al. Learning to diagnose cirrhosis with liver capsule guided ultrasound image classification[J]. Sensors (Basel), 2017, 17(1): 149. DOI: 10.3390/s17010149. [6] SCHMAUCH B, HERENT P, JEHANNO P, et al. Diagnosis of focal liver lesions from ultrasound using deep learning[J]. Diagn Interv Imaging, 2019, 100(4): 227-233. DOI: 10.1016/j.diii.2019.02.009. [7] GUO LH, WANG D, QIAN YY, et al. A two-stage multi-view learning framework based computer-aided diagnosis of liver tumors with contrast enhanced ultrasound images[J]. Clin Hemorheol Microcirc, 2018, 69(3): 343-354. DOI: 10.3233/CH-170275. [8] MOKRANE FZ, LU L, VAVASSEUR A, et al. Radiomics machine-learning signature for diagnosis of hepatocellular carcinoma in cirrhotic patients with indeterminate liver nodules[J]. Eur Radiol, 2020, 30(1): 558-570. DOI: 10.1007/s00330-019-06347-w. [9] YASAKA K, AKAI H, ABE O, et al. Deep learning with convolutional neural network for differentiation of liver masses at dynamic contrast-enhanced CT: A preliminary study[J]. Radiology, 2018, 286(3): 887-896. DOI: 10.1148/radiol.2017170706. [10] VIVANTI R, SZESKIN A, LEV-COHAIN N, et al. Automatic detection of new tumors and tumor burden evaluation in longitudinal liver CT scan studies[J]. Int J Comput Assist Radiol Surg, 2017, 12(11): 1945-1957. DOI: 10.1007/s11548-017-1660-z. [11] PREIS O, BLAKE MA, SCOTT JA. Neural network evaluation of PET scans of the liver: A potentially useful adjunct in clinical interpretation[J]. Radiology, 2011, 258(3): 714-721. DOI: 10.1148/radiol.10100547. [12] KIANI A, UYUMAZTURK B, RAJPURKAR P, et al. Impact of a deep learning assistant on the histopathologic classification of liver cancer[J]. NPJ Digit Med, 2020, 3: 23. DOI: 10.1038/s41746-020-0232-8. [13] LIAO H, XIONG T, PENG J, et al. Classification and prognosis prediction from histopathological images of hepatocellular carcinoma by a fully automated pipeline based on machine learning[J]. Ann Surg Oncol, 2020, 27(7): 2359-2369. DOI: 10.1245/s10434-019-08190-1. [14] CAI W, HE B, HU M, et al. A radiomics-based nomogram for the preoperative prediction of posthepatectomy liver failure in patients with hepatocellular carcinoma[J]. Surg Oncol, 2019, 28: 78-85. DOI: 10.1016/j.suronc.2018.11.013. [15] FORNER A, REIG M, BRUIX J. Hepatocellular carcinoma[J]. Lancet, 2018, 391(10127): 1301-1314. DOI: 10.1016/S0140-6736(18)30010-2. [16] MA X, WEI J, GU D, et al. Preoperative radiomics nomogram for microvascular invasion prediction in hepatocellular carcinoma using contrast-enhanced CT[J]. Eur Radiol, 2019, 29(7): 3595-3605. DOI: 10.1007/s00330-018-5985-y. [17] ZHOU W, ZHANG L, WANG K, et al. Malignancy characterization of hepatocellular carcinomas based on texture analysis of contrast-enhanced MR images[J]. J Magn Reson Imaging, 2017, 45(5): 1476-1484. DOI: 10.1002/jmri.25454. [18] DONG Y, ZHOU L, XIA W, et al. Preoperative prediction of microvascular invasion in hepatocellular carcinoma: Initial application of a radiomic algorithm based on grayscale ultrasound images[J]. Front Oncol, 2020, 10: 353. DOI: 10.3389/fonc.2020.00353. [19] JI GW, ZHU FP, XU Q, et al. Machine-learning analysis of contrast-enhanced CT radiomics predicts recurrence of hepatocellular carcinoma after resection: A multi-institutional study[J]. EBioMedicine, 2019, 50: 156-165. DOI: 10.1016/j.ebiom.2019.10.057. [20] SAILLARD C, SCHMAUCH B, LAIFA O, et al. Predicting survival after hepatocellular carcinoma resection using deep learning on histological slides[J]. Hepatology, 2020, 72(6): 2000-2013. DOI: 10.1002/hep.31207. [21] SCHOENBERG MB, BUCHER JN, KOCH D, et al. A novel machine learning algorithm to predict disease free survival after resection of hepatocellular carcinoma[J]. Ann Transl Med, 2020, 8(7): 434. DOI: 10.21037/atm.2020.04.16. [22] MORSHID A, ELSAYES KM, KHALAF AM, et al. A machine learning model to predict hepatocellular carcinoma response to transcatheter arterial chemoembolization[J]. Radiol Artif Intell, 2019, 1(5): e180021. DOI: 10.1148/ryai.2019180021. [23] PENG J, KANG S, NING Z, et al. Residual convolutional neural network for predicting response of transarterial chemoembolization in hepatocellular carcinoma from CT imaging[J]. Eur Radiol, 2020, 30(1): 413-424. DOI: 10.1007/s00330-019-06318-1. [24] LIU D, LIU F, XIE X, et al. Accurate prediction of responses to transarterial chemoembolization for patients with hepatocellular carcinoma by using artificial intelligence in contrast-enhanced ultrasound[J]. Eur Radiol, 2020, 30(4): 2365-2376. DOI: 10.1007/s00330-019-06553-6. [25] ABAJIAN A, MURALI N, SAVIC LJ, et al. Predicting treatment response to image-guided therapies using machine learning: An example for trans-arterial treatment of hepatocellular carcinoma[J]. J Vis Exp, 2018, (140): 58382. DOI: 10.3791/58382. [26] CESARETTI M, BRUSTIA R, GOUMARD C, et al. Use of artificial intelligence as an innovative method for liver graft macrosteatosis assessment[J]. Liver Transpl, 2020, 26(10): 1224-1232. DOI: 10.1002/lt.25801. [27] NAM JY, LEE JH, BAE J, et al. Novel model to predict HCC recurrence after liver transplantation obtained using deep learning: A multicenter study[J]. Cancers (Basel), 2020, 12(10): 2791. DOI: 10.3390/cancers12102791. [28] MOLINARI M, AYLOO S, TSUNG A, et al. Prediction of perioperative mortality of cadaveric liver transplant recipients during their evaluations[J]. Transplantation, 2019, 103(10): e297-e307. DOI: 10.1097/TP.0000000000002810. [29] BRICEÑO J, CRUZ-RAMÍREZ M, PRIETO M, et al. Use of artificial intelligence as an innovative donor-recipient matching model for liver transplantation: Results from a multicenter Spanish study[J]. J Hepatol, 2014, 61(5): 1020-1028. DOI: 10.1016/j.jhep.2014.05.039. [30] BERTSIMAS D, KUNG J, TRICHAKIS N, et al. Development and validation of an optimized prediction of mortality for candidates awaiting liver transplantation[J]. Am J Transplant, 2019, 19(4): 1109-1118. DOI: 10.1111/ajt.15172. [31] TAN YF, ZHANG W, YANG JY, et al. Application of artificial intelligence in diagnosis and treatment of liver cancer[J]. Chin J Bases Clin Gen Surg, 2020, 27(9): 1057-1061. DOI: 10.7507/1007-9424.202008023.谭一非, 章蔚, 杨家印, 等. 人工智能在肝癌诊治中的应用进展[J]. 中国普外基础与临床杂志, 2020, 27(9): 1057-1061. DOI: 10.7507/1007-9424.202008023. [32] VILLANUEVA A. Hepatocellular carcinoma[J]. N Engl J Med, 2019, 380(15): 1450-1462. DOI: 10.1056/NEJMra1713263. [33] CITONE M, FANELLI F, FALCONE G, et al. A closer look to the new frontier of artificial intelligence in the percutaneous treatment of primary lesions of the liver[J]. Med Oncol, 2020, 37(6): 55. DOI: 10.1007/s12032-020-01380-y. [34] AHN SJ, LEE JM, LEE DH, et al. Real-time US-CT/MR fusion imaging for percutaneous radiofrequency ablation of hepatocellular carcinoma[J]. J Hepatol, 2017, 66(2): 347-354. DOI: 10.1016/j.jhep.2016.09.003. [35] HUANG Q, ZENG Q, LONG Y, et al. Fusion imaging techniques and contrast-enhanced ultrasound for thermal ablation of hepatocellular carcinoma - A prospective randomized controlled trial[J]. Int J Hyperthermia, 2019, 36(1): 1207-1215. DOI: 10.1080/02656736.2019.1687945. [36] YANG WL, LEE YE, CHEN MH, et al. In-silico drug screening and potential target identification for hepatocellular carcinoma using Support Vector Machines based on drug screening result[J]. Gene, 2013, 518(1): 201-208. DOI: 10.1016/j.gene.2012.11.030. [37] SHI B, WANG JL. Research advances in artificial intelligence in predicting prognosis of patients with hepatocellular carcinoma[J]. Acad J Chinese PLA Postgrad Med Sch, 2020, 41(9): 922-925. DOI: 10.3969/j.issn.2095-5227.2020.09.017.史斌, 王建立. 人工智能对肝癌患者预后预测的研究进展[J]. 解放军医学院学报, 2020, 41(9): 922-925. DOI: 10.3969/j.issn.2095-5227.2020.09.017. -
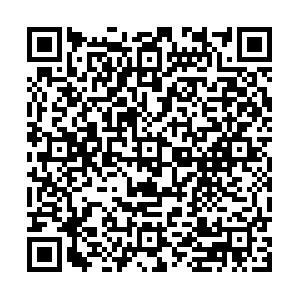
计量
- 文章访问数: 1135
- HTML全文浏览量: 181
- PDF下载量: 160
- 被引次数: 0