人工智能在肝移植中的应用
DOI: 10.3969/j.issn.1001-5256.2022.01.005
利益冲突声明:所有作者均声明不存在利益冲突。
作者贡献声明:梁智星负责撰写论文;叶林森负责修改论文;杨扬负责拟定写作思路,指导撰写文章并最后定稿。
Application of artificial intelligence in liver transplantation
-
摘要: 随着5G和大数据时代的来临, 多维度、大样本的复杂医疗数据为新时代临床医学带来众多机遇和挑战。与传统方法相比, 人工智能可以更高效地挖掘庞大数据集中的隐藏联系, 越来越多的学者将这一先进技术应用于疾病的诊断和治疗。经过半个多世纪的发展和完善, 肝移植已成为治疗各种终末期肝病最有效的方法。与其他领域的“单患者”数据分析不同, 肝移植通常需要考虑供体和受体“双患者”的特征以及移植过程中的变量, 产生较其他疾病更为庞大的医疗数据, 这与人工智能的优势尤为契合。有效利用人工智能的手段、结合临床研究开展将为智能精准临床医学时代拉开新的序幕。对于人工智能在肝移植的交叉应用, 需要全面讨论人工智能技术在肝移植中的优势和局限性, 并提出该领域的未来方向。Abstract: With the advent of the era of 5G and big data, complex medical data with multiple dimensions and a large sample size bring both opportunities and challenges for clinical medicine in the new era. Compared with conventional methods, artificial intelligence can detect the hidden patterns within large datasets, and more and more scholars are applying such advanced technology in the diagnosis and treatment of diseases. After development and perfection for more than half a century, liver transplantation has become the most effective treatment method for end-stage liver diseases. Unlike the analysis of "single-patient" data in other fields, liver transplantation usually requires the consideration of the features of both the donor and the recipient and the variables during transplantation, thus generating a larger volume of medical data than other diseases, which is particularly in line with the advantages of artificial intelligence. Effective application of artificial intelligence and its combination with clinical research will usher in the new era of precision medicine. The advantages and limitations of artificial intelligence technology should be comprehensively discussed for the cross-application of artificial intelligence in liver transplantation, and the future directions of this field should also be proposed.
-
Key words:
- Liver Transplantation /
- Artificial Intelligence /
- Machine Learning /
- Deep Learning
-
表 1 常用的AI算法
名称 核心原理 优点 缺点 应用 传统ML监督算法 逻辑回归 使用logistic函数对分类因变量建模 实现简单;计算量小, 对算力要求不高;易于解释各特征比重 容易欠拟合, 准确度欠佳, 不能很好地处理大量多类特征或变量 临床事件预测, 疾病分类, 以往最常用的生物统计学方法 朴素贝叶斯 基于贝叶斯定理的分类器, 即先验概率+数据=后验概率 适用于小规模数据;对缺失数据不敏感 假设变量间相互独立(往往不成立), 需要知道先验概率 临床事件预测疾病分类 支持向量机 利用核函数对高维数据进行非线性分类 适用于高维、非线性数据, 泛化能力好 算力要求高, 可解释性不佳, 对缺失数据敏感 风险预测, 疾病分类, 图像识别 K近邻算法 基于向量间欧氏距离, 将目标以最接近的K个邻近值来代表分类 适用于非线性、多分类, 准确度高 算力要求高, 对数据依赖度高, 对多维数据处理不佳 临床事件预测, 疾病分类 决策树 利用树状模型回答是/否问题以预测可能结果 可解释性强, 无需研究领域知识, 对缺失值不敏感 容易过拟合, 忽略变量间的相关性 临床事件预测, 疾病分类 随机森林 集成多个决策树输出结果, 以最多的结果为最终的输出 适用于高维数据, 准确度较决策树高 容易过拟合, 可解释性一般 临床事件预测, 疾病分类 梯度提升方法 依次迭代多个较弱的预测模型, 结合得到更佳的输出 高准确性, 无需特征筛选 算力要求高, 对离群值敏感 临床事件预测, 疾病分类 神经网络与DL ANN 多个神经元模型连接构成, 各层神经元间可传递信息 适用于大规模数据集, 挖掘复杂、非线性关系 算力要求很高, “黑箱”模型, 可解释性差 风险预测, 疾病分类 CNN 模拟动物视觉皮层连接模式的深度学习模型 适用于图像类数据集, 自动提取图像空间特征 算力要求很高, 每层输出物理含义不明确 图像识别, 影像诊断 RNN 模拟信号在神经回路中循环传递, 具有“记忆功能” 特别适用于序列数据集 算力要求很高;建模训练难度大 语音/手写体识别临床事件预测 注:ANN, 人工神经网络;CNN, 卷层神经网络;RNN, 循环神经网络。 -
[1] PASTORINO R, de VITO C, MIGLIARA G, et al. Benefits and challenges of Big Data in healthcare: An overview of the European initiatives[J]. Eur J Public Health, 2019, 29(Supplement_3): 23-27. DOI: 10.1093/eurpub/ckz168. [2] TRAN BX, VU GT, HA GH, et al. Global evolution of research in artificial intelligence in health and medicine: A bibliometric study[J]. J Clin Med, 2019, 8(3): 360. DOI: 10.3390/jcm8030360. [3] DARCY AM, LOUIE AK, ROBERTS LW. Machine learning and the profession of medicine[J]. JAMA, 2016, 315(6): 551-552. DOI: 10.1001/jama.2015.18421. [4] ESTEVA A, ROBICQUET A, RAMSUNDAR B, et al. A guide to deep learning in healthcare[J]. Nat Med, 2019, 25(1): 24-29. DOI: 10.1038/s41591-018-0316-z. [5] MCCORMACK L, PETROWSKY H, JOCHUM W, et al. Use of severely steatotic grafts in liver transplantation: A matched case-control study[J]. Ann Surg, 2007, 246(6): 940-946; discussion 946-948. DOI: 10.1097/SLA.0b013e31815c2a3f. [6] VOLK ML, RONEY M, MERION RM. Systematic bias in surgeons' predictions of the donor-specific risk of liver transplant graft failure[J]. Liver Transpl, 2013, 19(9): 987-990. DOI: 10.1002/lt.23683. [7] KUPPILI V, BISWAS M, SREEKUMAR A, et al. Extreme learning machine framework for risk stratification of fatty liver disease using ultrasound tissue characterization[J]. J Med Syst, 2017, 41(10): 152. DOI: 10.1007/s10916-017-0797-1. [8] BYRA M, STYCZYNSKI G, SZMIGIELSKI C, et al. Transfer learning with deep convolutional neural network for liver steatosis assessment in ultrasound images[J]. Int J Comput Assist Radiol Surg, 2018, 13(12): 1895-1903. DOI: 10.1007/s11548-018-1843-2. [9] VANDERBECK S, BOCKHORST J, KOMOROWSKI R, et al. Automatic classification of white regions in liver biopsies by supervised machine learning[J]. Hum Pathol, 2014, 45(4): 785-792. DOI: 10.1016/j.humpath.2013.11.011. [10] MOCCIA S, MATTOS LS, PATRINI I, et al. Computer-assisted liver graft steatosis assessment via learning-based texture analysis[J]. Int J Comput Assist Radiol Surg, 2018, 13(9): 1357-1367. DOI: 10.1007/s11548-018-1787-6. [11] CESARETTI M, BRUSTIA R, GOUMARD C, et al. Use of artificial intelligence as an innovative method for liver graft macrosteatosis assessment[J]. Liver Transpl, 2020, 26(10): 1224-1232. DOI: 10.1002/lt.25801. [12] CROOME KP, MAROTTA P, WALL WJ, et al. Should a lower quality organ go to the least sick patient? Model for end-stage liver disease score and donor risk index as predictors of early allograft dysfunction[J]. Transplant Proc, 2012, 44(5): 1303-1306. DOI: 10.1016/j.transproceed.2012.01.115. [13] BRICEÑO J, CRUZ-RAMÍREZ M, PRIETO M, et al. Use of artificial intelligence as an innovative donor-recipient matching model for liver transplantation: Results from a multicenter Spanish study[J]. J Hepatol, 2014, 61(5): 1020-1028. DOI: 10.1016/j.jhep.2014.05.039. [14] BRICEÑO J, AYLLÓN MD, CIRIA R. Machine-learning algorithms for predicting results in liver transplantation: The problem of donor-recipient matching[J]. Curr Opin Organ Transplant, 2020, 25(4): 406-411. DOI: 10.1097/MOT.0000000000000781. [16] DORADO-MORENO M, PÉREZ-ORTIZ M, GUTIÉRREZ PA, et al. Dynamically weighted evolutionary ordinal neural network for solving an imbalanced liver transplantation problem[J]. Artif Intell Med, 2017, 77: 1-11. DOI: 10.1016/j.artmed.2017.02.004. [17] CRUZ-RAMÍREZ M, HERVÁS-MARTÍNEZ C, FERNÁNDEZ JC, et al. Predicting patient survival after liver transplantation using evolutionary multi-objective artificial neural networks[J]. Artif Intell Med, 2013, 58(1): 37-49. DOI: 10.1016/j.artmed.2013.02.004. [18] BERTSIMAS D, KUNG J, TRICHAKIS N, et al. Development and validation of an optimized prediction of mortality for candidates awaiting liver transplantation[J]. Am J Transplant, 2019, 19(4): 1109-1118. DOI: 10.1111/ajt.15172. [19] GUIJO-RUBIO D, BRICEÑO J, GUTIÉRREZ PA, et al. Statistical methods versus machine learning techniques for donor-recipient matching in liver transplantation[J]. PLoS One, 2021, 16(5): e0252068. DOI: 10.1371/journal.pone.0252068. [20] VAGEFI PA, BERTSIMAS D, HIROSE R, et al. The rise and fall of the model for end-stage liver disease score and the need for an optimized machine learning approach for liver allocation[J]. Curr Opin Organ Transplant, 2020, 25(2): 122-125. DOI: 10.1097/MOT.0000000000000734. [21] WINGFIELD LR, CERESA C, THOROGOOD S, et al. Using artificial intelligence for predicting survival of individual grafts in liver transplantation: A systematic review[J]. Liver Transpl, 2020, 26(7): 922-934. DOI: 10.1002/lt.25772. [22] LIU CL, SOONG RS, LEE WC, et al. Predicting short-term survival after liver transplantation using machine learning[J]. Sci Rep, 2020, 10(1): 5654. DOI: 10.1038/s41598-020-62387-z. [23] CHEN C, YANG D, GAO S, et al. Development and performance assessment of novel machine learning models to predict pneumonia after liver transplantation[J]. Respir Res, 2021, 22(1): 94. DOI: 10.1186/s12931-021-01690-3. [24] HOOT N, ARONSKY D. Using Bayesian networks to predict survival of liver transplant patients[J]. AMIA Annu Symp Proc, 2005, 2005: 345-349. [25] KANTIDAKIS G, PUTTER H, LANCIA C, et al. Survival prediction models since liver transplantation-comparisons between Cox models and machine learning techniques[J]. BMC Med Res Methodol, 2020, 20(1): 277. DOI: 10.1186/s12874-020-01153-1. [26] ZHANG M, YIN F, CHEN B, et al. Mortality risk after liver transplantation in hepatocellular carcinoma recipients: A nonlinear predictive model[J]. Surgery, 2012, 151(6): 889-897. DOI: 10.1016/j.surg.2011.12.034. [28] LAU L, KANKANIGE Y, RUBINSTEIN B, et al. Machine-learning algorithms predict graft failure after liver transplantation[J]. Transplantation, 2017, 101(4): e125-e132. DOI: 10.1097/TP.0000000000001600. [29] BHAT V, TAZARI M, WATT KD, et al. New-onset diabetes and preexisting diabetes are associated with comparable reduction in long-term survival after liver transplant: A machine learning approach[J]. Mayo Clin Proc, 2018, 93(12): 1794-1802. DOI: 10.1016/j.mayocp.2018.06.020. [30] ANDRES A, MONTANO-LOZA A, GREINER R, et al. A novel learning algorithm to predict individual survival after liver transplantation for primary sclerosing cholangitis[J]. PLoS One, 2018, 13(3): e0193523. DOI: 10.1371/journal.pone.0193523. [31] WADHWANI SI, HSU EK, SHAFFER ML, et al. Predicting ideal outcome after pediatric liver transplantation: An exploratory study using machine learning analyses to leverage studies of pediatric liver transplantation data[J]. Pediatr Transplant, 2019, 23(7): e13554. DOI: 10.1111/petr.13554. [32] YASODHARA A, DONG V, AZHIE A, et al. Identifying modifiable predictors of long-term survival in liver transplant recipients with diabetes mellitus using machine learning[J]. Liver Transpl, 2021, 27(4): 536-547. DOI: 10.1002/lt.25930. [33] JAIN V, BANSAL A, RADAKOVICH N, et al. Machine learning models to predict major adverse cardiovascular events after orthotopic liver transplantation: A cohort study[J]. J Cardiothorac Vasc Anesth, 2021, 35(7): 2063-2069. DOI: 10.1053/j.jvca.2021.02.006. [34] ZHANG Y, YANG D, LIU Z, et al. An explainable supervised machine learning predictor of acute kidney injury after adult deceased donor liver transplantation[J]. J Transl Med, 2021, 19(1): 321. DOI: 10.1186/s12967-021-02990-4. [35] JIANG YQ, CAO SE, CAO S, et al. Preoperative identification of microvascular invasion in hepatocellular carcinoma by XGBoost and deep learning[J]. J Cancer Res Clin Oncol, 2021, 147(3): 821-833. DOI: 10.1007/s00432-020-03366-9. [36] AKBILGIC O, DAVIS RL. The promise of machine learning: When will it be delivered?[J]. J Card Fail, 2019, 25(6): 484-485. DOI: 10.1016/j.cardfail.2019.04.006. [37] CARUANA R, LOU Y, GEHRKE J, et al. Intelligible models for healthcare: Predicting pneumonia risk and hospital 30-day readmission[C]. ACM, 2015. -
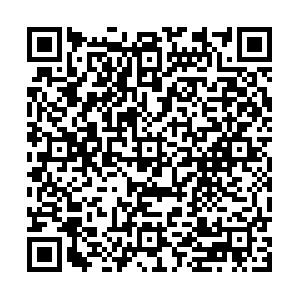
表(1)
计量
- 文章访问数: 794
- HTML全文浏览量: 226
- PDF下载量: 116
- 被引次数: 0