基于人工智能的肝细胞癌精准影像学诊断和复发预测
DOI: 10.3969/j.issn.1001-5256.2022.03.006
利益冲突声明: 所有作者均声明不存在利益冲突。
作者贡献声明: 刘一萍、李新平、夏金菊、宋凯荣负责文献收集与总结; 陈磊、刘婉敏及贾宁阳负责文章攥写及修改。
Accurate imaging diagnosis and recurrence prediction of hepatocellular carcinoma based on artificial intelligence
-
摘要: 人工智能在医疗领域的融合发展迅速,特别在影像医学的诊断、治疗和疗效评估等方面有突破性进展。本文回顾了人工智能在肝细胞癌影像学诊断及其结合临床特征进行疗效评估和预后预测的效能方面的研究进展,展望了在日益增长的临床需求与快速进步的诊疗技术时代,如何将人工智能更好地运用于肝细胞癌影像学实践中。Abstract: The integration of artificial intelligence into the medical field is developing rapidly and has achieved ground-breaking advances in the diagnosis, treatment, and efficacy evaluation of imaging medicine. This article reviews the research advances in artificial intelligence in imaging diagnosis of hepatocellular carcinoma and its performance in evaluating treatment outcome and predicting prognosis in combination with clinical features and looks forward to how artificial intelligence can be better used in the practice of hepatocellular carcinoma imaging in the era of growing clinical needs and rapid advances in diagnosis and treatment techniques.
-
Key words:
- Hepatocellular Carcinoma /
- Diagnostic Imaging /
- Recurrence /
- Forecasting
-
[1] MARRERO JA, KULIK LM, SIRLIN CB, et al. Diagnosis, staging, and management of hepatocellular carcinoma: 2018 practice guidance by the American Association for the Study of Liver Diseases[J]. Hepatology, 2018, 68(2): 723-750. DOI: 10.1002/hep.29913. [2] KAMBADAKONE AR, FUNG A, GUPTA RT, et al. Correction to: LI-RADS technical requirements for CT, MRI, and contrast-enhanced ultrasound[J]. Abdom Radiol (NY), 2018, 43(1): 240. DOI: 10.1007/s00261-017-1345-7. [3] JIANG H, LIU X, CHEN J, et al. Man or machine? Prospective comparison of the version 2018 EASL, LI-RADS criteria and a radiomics model to diagnose hepatocellular carcinoma[J]. Cancer Imaging, 2019, 19(1): 84. DOI: 10.1186/s40644-019-0266-9. [4] HEIMBACH JK, KULIK LM, FINN RS, et al. AASLD guidelines for the treatment of hepatocellular carcinoma[J]. Hepatology, 2018, 67(1): 358-380. DOI: 10.1002/hep.29086. [5] CUOCOLO R, CARUSO M, PERILLO T, et al. Machine learning in oncology: A clinical appraisal[J]. Cancer Lett, 2020, 481: 55-62. DOI: 10.1016/j.canlet.2020.03.032. [6] HU W, YANG H, XU H, et al. Radiomics based on artificial intelligence in liver diseases: Where we are?[J]. Gastroenterol Rep(Oxf), 2020, 8(2): 90-97. DOI: 10.1093/gastro/goaa011. [7] LANGLOTZ CP, ALLEN B, ERICKSON BJ, et al. A roadmap for foundational research on artificial intelligence in medical imaging: From the 2018 NIH/RSNA/ACR/The Academy Workshop[J]. Radiology, 2019, 291(3): 781-791. DOI: 10.1148/radiol.2019190613. [8] SAVADJIEV P, CHONG J, DOHAN A, et al. Demystification of AI-driven medical image interpretation: Past, present and future[J]. Eur Radiol, 2019, 29(3): 1616-1624. DOI: 10.1007/s00330-018-5674-x. [9] YAMADA A, OYAMA K, FUJITA S, et al. Dynamic contrast-enhanced computed tomography diagnosis of primary liver cancers using transfer learning of pretrained convolutional neural networks: Is registration of multiphasic images necessary?[J]. Int J Comput Assist Radiol Surg, 2019, 14(8): 1295-1301. DOI: 10.1007/s11548-019-01987-1. [10] CHOY G, KHALILZADEH O, MICHALSKI M, et al. Current applications and future impact of machine learning in radiology[J]. Radiology, 2018, 288(2): 318-328. DOI: 10.1148/radiol.2018171820. [11] CHARTRAND G, CHENG PM, VORONTSOV E, et al. Deep learning: A primer for radiologists[J]. Radiographics, 2017, 37(7): 2113-2131. DOI: 10.1148/rg.2017170077. [12] FENG ST, JIA Y, LIAO B, et al. Preoperative prediction of microvascular invasion in hepatocellular cancer: A radiomics model using Gd-EOB-DTPA-enhanced MRI[J]. Eur Radiol, 2019, 29(9): 4648-4659. DOI: 10.1007/s00330-018-5935-8. [13] HARDING-THEOBALD E, LOUISSAINT J, MARAJ B, et al. Systematic review: Radiomics for the diagnosis and prognosis of hepatocellular carcinoma[J]. Aliment Pharmacol Ther, 2021, 54(7): 890-901. DOI: 10.1111/apt.16563. [14] JIMÉNEZ PÉREZ M, GRANDE RG. Application of artificial intelligence in the diagnosis and treatment of hepatocellular carcinoma: A review[J]. World J Gastroenterol, 2020, 26(37): 5617-5628. DOI: 10.3748/wjg.v26.i37.5617. [15] WAKABAYASHI T, OUHMICH F, GONZALEZ-CABRERA C, et al. Radiomics in hepatocellular carcinoma: A quantitative review[J]. Hepatol Int, 2019, 13(5): 546-559. DOI: 10.1007/s12072-019-09973-0. [16] MA X, WEI J, GU D, et al. Preoperative radiomics nomogram for microvascular invasion prediction in hepatocellular carcinoma using contrast-enhanced CT[J]. Eur Radiol, 2019, 29(7): 3595-3605. DOI: 10.1007/s00330-018-5985-y. [17] HECTORS SJ, LEWIS S, BESA C, et al. MRI radiomics features predict immuno-oncological characteristics of hepatocellular carcinoma[J]. Eur Radiol, 2020, 30(7): 3759-3769. DOI: 10.1007/s00330-020-06675-2. [18] WEI J, JIANG H, GU D, et al. Radiomics in liver diseases: Current progress and future opportunities[J]. Liver Int, 2020, 40(9): 2050-2063. DOI: 10.1111/liv.14555. [19] LEWIS S, HECTORS S, TAOULI B. Radiomics of hepatocellular carcinoma[J]. Abdom Radiol (NY), 2021, 46(1): 111-123. DOI: 10.1007/s00261-019-02378-5. [20] CUOCOLO R, COMELLI A, STEFANO A, et al. Deep learning whole- gland and zonal prostate segmentation on a public MRI dataset[J]. J Magn Reson Imaging, 2021, 54(2): 452-459. DOI: 10.1002/jmri.27585. [21] NAYAK A, BAIDYA KAYAL E, ARYA M, et al. Computer-aided diagnosis of cirrhosis and hepatocellular carcinoma using multi-phase abdomen CT[J]. Int J Comput Assist Radiol Surg, 2019, 14(8): 1341-1352. DOI: 10.1007/s11548-019-01991-5. [22] WARDHANA G, NAGHIBI H, SIRMACEK B, et al. Toward reliable automatic liver and tumor segmentation using convolutional neural network based on 2.5D models[J]. Int J Comput Assist Radiol Surg, 2021, 16(1): 41-51. DOI: 10.1007/s11548-020-02292-y. [23] OUHMICH F, AGNUS V, NOBLET V, et al. Liver tissue segmentation in multiphase CT scans using cascaded convolutional neural networks[J]. Int J Comput Assist Radiol Surg, 2019, 14(8): 1275-1284. DOI: 10.1007/s11548-019-01989-z. [24] BOUSABARAH K, LETZEN B, TEFERA J, et al. Automated detection and delineation of hepatocellular carcinoma on multiphasic contrast-enhanced MRI using deep learning[J]. Abdom Radiol (NY), 2021, 46(1): 216-225. DOI: 10.1007/s00261-020-02604-5. [25] ZHEN SH, CHENG M, TAO YB, et al. Deep learning for accurate diagnosis of liver tumor based on magnetic resonance imaging and clinical data[J]. Front Oncol, 2020, 10: 680. DOI: 10.3389/fonc.2020.00680. [26] JANSEN M, KUIJF HJ, VELDHUIS WB, et al. Automatic classification of focal liver lesions based on MRI and risk factors[J]. PLoS One, 2019, 14(5): e0217053. DOI: 10.1371/journal.pone.0217053. [27] MOKRANE FZ, LU L, VAVASSEUR A, et al. Radiomics machine-learning signature for diagnosis of hepatocellular carcinoma in cirrhotic patients with indeterminate liver nodules[J]. Eur Radiol, 2020, 30(1): 558-570. DOI: 10.1007/s00330-019-06347-w. [28] WANG M, FU F, ZHENG B, et al. Development of an AI system for accurately diagnose hepatocellular carcinoma from computed tomography imaging data[J]. Br J Cancer, 2021, 125(8): 1111-1121. DOI: 10.1038/s41416-021-01511-w. [29] OESTMANN PM, WANG CJ, SAVIC LJ, et al. Deep learning-assisted differentiation of pathologically proven atypical and typical hepatocellular carcinoma (HCC) versus non-HCC on contrast-enhanced MRI of the liver[J]. Eur Radiol, 2021, 31(7): 4981-4990. DOI: 10.1007/s00330-020-07559-1. [30] VIVANTI R, JOSKOWICZ L, LEV-COHAIN N, et al. Patient-specific and global convolutional neural networks for robust automatic liver tumor delineation in follow-up CT studies[J]. Med Biol Eng Comput, 2018, 56(9): 1699-1713. DOI: 10.1007/s11517-018-1803-6. [31] SHI W, KUANG S, CAO S, et al. Deep learning assisted differentiation of hepatocellular carcinoma from focal liver lesions: Choice of four-phase and three-phase CT imaging protocol[J]. Abdom Radiol (NY), 2020, 45(9): 2688-2697. DOI: 10.1007/s00261-020-02485-8. [32] HAMM CA, WANG CJ, SAVIC LJ, et al. Deep learning for liver tumor diagnosis part I: Development of a convolutional neural network classifier for multi-phasic MRI[J]. Eur Radiol, 2019, 29(7): 3338-3347. DOI: 10.1007/s00330-019-06205-9. [33] WU Y, WHITE GM, CORNELIUS T, et al. Deep learning LI-RADS grading system based on contrast enhanced multiphase MRI for differentiation between LR-3 and LR-4/LR-5 liver tumors[J]. Ann Transl Med, 2020, 8(11): 701. DOI: 10.21037/atm.2019.12.151. [34] MAO B, ZHANG L, NING P, et al. Preoperative prediction for pathological grade of hepatocellular carcinoma via machine learning-based radiomics[J]. Eur Radiol, 2020, 30(12): 6924-6932. DOI: 10.1007/s00330-020-07056-5. [35] WU M, TAN H, GAO F, et al. Predicting the grade of hepatocellular carcinoma based on non-contrast-enhanced MRI radiomics signature[J]. Eur Radiol, 2019, 29(6): 2802-2811. DOI: 10.1007/s00330-018-5787-2. [36] CHEN Y, XIA Y, TOLAT PP, et al. Comparison of conventional gadoxetate disodium-enhanced MRI features and radiomics signatures with machine learning for diagnosing microvascular invasion[J]. AJR Am J Roentgenol, 2021, 216(6): 1510-1520. DOI: 10.2214/AJR.20.23255. [37] BORHANI AA, CATANIA R, VELICHKO YS, et al. Radiomics of hepatocellular carcinoma: Promising roles in patient selection, prediction, and assessment of treatment response[J]. Abdom Radiol (NY), 2021, 46(8): 3674-3685. DOI: 10.1007/s00261-021-03085-w. [38] LAI Q, VITALE A, HALAZUN K, et al. Identification of an upper limit of tumor burden for downstaging in candidates with hepatocellular cancer waiting for liver transplantation: A west-east collaborative effort[J]. Cancers (Basel), 2020, 12(2): 452. DOI: 10.3390/cancers12020452. [39] HUANG S, YANG J, FONG S, et al. Artificial intelligence in cancer diagnosis and prognosis: Opportunities and challenges[J]. Cancer Lett, 2020, 471: 61-71. DOI: 10.1016/j.canlet.2019.12.007. [40] LAI Q, SPOLETINI G, MENNINI G, et al. Prognostic role of artificial intelligence among patients with hepatocellular cancer: A systematic review[J]. World J Gastroenterol, 2020, 26(42): 6679-6688. DOI: 10.3748/wjg.v26.i42.6679. [41] GUO D, GU D, WANG H, et al. Radiomics analysis enables recurrence prediction for hepatocellular carcinoma after liver transplantation[J]. Eur J Radiol, 2019, 117: 33-40. DOI: 10.1016/j.ejrad.2019.05.010. [42] JI GW, ZHU FP, XU Q, et al. Machine-learning analysis of contrast-enhanced CT radiomics predicts recurrence of hepatocellular carcinoma after resection: A multi-institutional study[J]. EBioMedicine, 2019, 50: 156-165. DOI: 10.1016/j.ebiom.2019.10.057. [43] ZHANG Z, CHEN J, JIANG H, et al. Gadoxetic acid-enhanced MRI radiomics signature: Prediction of clinical outcome in hepatocellular carcinoma after surgical resection[J]. Ann Transl Med, 2020, 8(14): 870. DOI: 10.21037/atm-20-3041. [44] YUAN C, WANG Z, GU D, et al. Prediction early recurrence of hepatocellular carcinoma eligible for curative ablation using a Radiomics nomogram[J]. Cancer Imaging, 2019, 19(1): 21. DOI: 10.1186/s40644-019-0207-7. [45] SHEN JX, ZHOU Q, CHEN ZH, et al. Longitudinal radiomics algorithm of posttreatment computed tomography images for early detecting recurrence of hepatocellular carcinoma after resection or ablation[J]. Transl Oncol, 2021, 14(1): 100866. DOI: 10.1016/j.tranon.2020.100866. [46] ABAJIAN A, MURALI N, SAVIC LJ, et al. Predicting treatment response to intra-arterial therapies for hepatocellular carcinoma with the use of supervised machine learning-an artificial intelligence concept[J]. J Vasc Interv Radiol, 2018, 29(6): 850-857. e1. DOI: 10.1016/j.jvir.2018.01.769. [47] PENG J, KANG S, NING Z, et al. Residual convolutional neural network for predicting response of transarterial chemoembolization in hepatocellular carcinoma from CT imaging[J]. Eur Radiol, 2020, 30(1): 413-424. DOI: 10.1007/s00330-019-06318-1. [48] ZHANG L, XIA W, YAN ZP, et al. Deep learning predicts overall survival of patients with unresectable hepatocellular carcinoma treated by transarterial chemoembolization plus sorafenib[J]. Front Oncol, 2020, 10: 593292. DOI: 10.3389/fonc.2020.593292. -
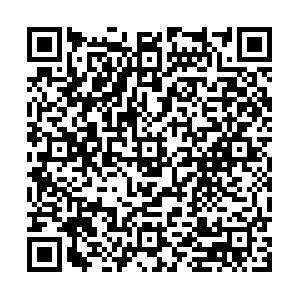
计量
- 文章访问数: 995
- HTML全文浏览量: 145
- PDF下载量: 162
- 被引次数: 0