机器学习在HBV感染相关疾病中的应用
DOI: 10.3969/j.issn.1001-5256.2021.07.044
Application of machine learning in hepatitis B virus-related liver diseases
-
摘要: 近年来,机器学习在医学领域的应用越来越广泛。在乳腺癌、糖尿病视网膜病变、神经精神疾病、动脉粥样硬化诊断和治疗方面均有新的进展。机器学习在肝病的诊断和预测方面具有很大潜力。结合患者血清学指标及影像学结果,利用机器学习的方法构建HBV相关肝病的诊断、预测模型,得到广泛的认可。旨在介绍机器学习方法在HBV相关肝病中的应用、现状、优点和进展。Abstract: Machine learning has been more and more widely used in the medical field in recent years, and new advances have been made in the diagnosis and treatment of breast cancer, diabetic retinopathy, neuropsychiatric diseases, and atherosclerosis. Machine learning is showing great potential in the diagnosis and prediction of liver diseases. With reference to patients' serological markers and imaging findings, the model established based on machine learning for the diagnosis and prediction of hepatitis B virus (HBV)-related liver diseases has been widely recognized. This article introduces the application, current status, advantages, and advances of machine learning in HBV-related liver diseases.
-
Key words:
- Machine Learning /
- Hepatitis B Virus /
- Liver Diseases
-
表 1 常见的算法列表的应用及优缺点
名称 应用 优缺点 SVM[3-4, 10] 是一种强大的分类工具,对数据进行二元分类的广义线性分类器,可以用于具有许多变量或维度的复杂数据集 优点:分离数据,最大化分离边际,提高了分类的敏感度和特异度,降低误分类率;
缺点:只考虑边缘的最大化而忽略了半径的最小化DT[11-12] 用于分类和回归的非参数监督学习方法,可以处理数值数据和分类数据 优点:可以对训练集产生良好的预测;
缺点:有许多分割树可能会过度拟合模型,从而导致测试集性能较差RF[8, 12] 用于分类和回归的典型集成学习方法 优点:可以弱化了单个决策树分类器经常出现的过拟合问题,对特征向量包含离散值的情况具有鲁棒性;
缺点:大量的树使算法速度慢,对实时预测无效NB[5, 8] 基于先验概率和观察到的训练集,通过计算最大后验概率预测给定样本分类 优点:结合先验概率和后验概率,避免了先验概率的主观偏见也避免了单独使用样本信息的过拟合线性;
缺点:先验概率很多时候取决于假设,可能导致预测模型不佳LR[5, 12] 广泛用于二元因变量的建模 优点:计算量与特征的数目相关,简单易理解,训练速度快;
缺点:形式简单,其准确率不高KNN[13-14] 广泛用于大数据分类,数据分布只涉及少量或不涉及先验知识的分类研究,KNN都是最佳选择 优点:精度高,简单好用,容易理解;
缺点:计算复杂性高,空间复杂性高,没有原则性的方法来选择使用的最近邻数,K值过高或过低都会产生不利的假阳性率或假阴性率MLP[6, 15] 是一种非参数动态模型,用于分类和回归 优点:可以使用大量的简单单元并行处理信息,并能够区分线性不可分的数据;
缺点:容易过拟合,参数难以调试,梯度弥散 -
[1] Chinese Society of Infectious Diseases, Chinese Medical Association; Chinese Society of Hepatology, Chinese Medical Association. Guidelines for the prevention and treatment of chronic hepatitis B (version 2019)[J]. J Clin Hepatol, 2019, 35(12): 2648-2669. DOI: 10.3969/1.issn. 1001-5256.2019.12.007.中华医学会感人病学分会, 中华医学会肝病学分会. 慢性乙型肝炎防治指南(2019年版)[J]. 临床肝胆病杂志, 2019, 35(12): 2648-2669. DOI: 10.3969/1.issn.1001-5256.2019.12.007. [2] LI W, HUANG Y, ZHUANG BW, et al. Multiparametric ultrasomics of significant liver fibrosis: A machine learning-based analysis[J]. Eur Radiol, 2019, 29(3): 1496-1506. DOI: 10.1007/s00330-018-5680-z. [3] HUANG S, CAI N, PACHECO PP, et al. Applications of Support Vector Machine (SVM) learning in cancer genomics[J]. Cancer Genomics Proteomics, 2018, 15(1): 41-51. DOI: 10.21873/cgp.20063. [4] HANDELMAN GS, KOK HK, CHANDRA RV, et al. eDoctor: Machine learning and the future of medicine[J]. J Intern Med, 2018, 284(6): 603-619. DOI: 10.1111/joim.12822. [5] DORADO-DÍAZ PI, SAMPEDRO-GÓMEZ J, VICENTE-PALACIOS V, et al. Applications of artificial intelligence in cardiology. The future is already here[J]. Rev Esp Cardiol (Engl Ed), 2019, 72(12): 1065-1075. DOI: 10.1016/j.rec.2019.05.014. [6] LAN X, WEI R, CAI HW, et al. Application of machine learning algorithm in medical field[J]. Chin Med Equipment J, 2019, 40 (3): 93-97. DOI: 10.19745/j.1003-8868.2019076.兰欣, 卫荣, 蔡宏伟, 等. 机器学习算法在医疗领域中的应用[J]. 医疗卫生装备, 2019, 40 (3): 93-97. DOI: 10.19745/j.1003-8868.2019076. [7] LIANG ST, GUO MZ, ZHAO LL, et al. Survey on medical decision support systems based on machine learning[J]. Comput Eng Applicat, 2019, 55(19): 1-11. DOI: 10.3778/j.issn.1002-8331.1903-0485.梁书彤, 郭茂祖, 赵玲玲. 基于机器学习的医疗决策支持系统综述[J]. 计算机工程与应用, 2019, 55 (19): 1-11. DOI: 10.3778/j.issn.1002-8331.1903-0485. [8] CHEN Y, LUO Y, HUANG W, et al. Machine-learning-based classification of real-time tissue elastography for hepatic fibrosis in patients with chronic hepatitis B[J]. Comput Biol Med, 2017, 89: 18-23. DOI: 10.1016/j.compbiomed.2017.07.012. [9] MA H, XU CF, SHEN Z, et al. Application of machine learning techniques for clinical predictive modeling: A cross-sectional study on nonalcoholic fatty liver disease in China[J]. Biomed Res Int, 2018, 2018: 4304376. DOI: 10.1155/2018/4304376. [10] WU X, ZUO W, LIN L, et al. F-SVM: Combination of feature transformation and SVM learning via convex relaxation[J]. IEEE Trans Neural Netw Learn Syst, 2018, 29(11): 5185-5199. DOI: 10.1109/TNNLS.2018.2791507. [11] de SANTANA FB, BORGES NETO W, POPPI RJ. Random forest as one-class classifier and infrared spectroscopy for food adulteration detection[J]. Food Chem, 2019, 293: 323-332. DOI: 10.1016/j.foodchem.2019.04.073. [12] TIAN X, CHONG Y, HUANG Y, et al. Using machine learning algorithms to predict hepatitis B surface antigen seroclearance[J]. Comput Math Methods Med, 2019, 2019: 6915850. DOI: 10.1155/2019/6915850. [13] ABU ALFEILAT HA, HASSANAT A, LASASSMEH O, et al. Effects of distance measure choice on K-nearest neighbor classifier performance: A review[J]. Big Data, 2019, 7(4): 221-248. DOI: 10.1089/big.2018.0175. [14] LO YC, RENSI SE, TORNG W, et al. Machine learning in chemoinformatics and drug discovery[J]. Drug Discov Today, 2018, 23(8): 1538-1546. DOI: 10.1016/j.drudis.2018.05.010. [15] WANG N, CAO Y, SONG W, et al. Serum peptide pattern that differentially diagnoses hepatitis B virus-related hepatocellular carcinoma from liver cirrhosis[J]. J Gastroenterol Hepatol, 2014, 29(7): 1544-1550. DOI: 10.1111/jgh.12545. [16] KHAN S, ULLAH R, KHAN A, et al. Analysis of hepatitis B virus infection in blood sera using Raman spectroscopy and machine learning[J]. Photodiagnosis Photodyn Ther, 2018, 23: 89-93. DOI: 10.1016/j.pdpdt.2018.05.010. [17] MUELLER-BRECKENRIDGE AJ, GARCIA-ALCALDE F, WILDUM S, et al. Machine-learning based patient classification using Hepatitis B virus full-length genome quasispecies from Asian and European cohorts[J]. Sci Rep, 2019, 9(1): 18892. DOI: 10.1038/s41598-019-55445-8. [18] ZHOU W, MA Y, ZHANG J, et al. Predictive model for inflammation grades of chronic hepatitis B: Large-scale analysis of clinical parameters and gene expressions[J]. Liver Int, 2017, 37(11): 1632-1641. DOI: 10.1111/liv.13427. [19] CAO Y, HE K, CHENG M, et al. Two classifiers based on serum peptide pattern for prediction of HBV-induced liver cirrhosis using MALDI-TOF MS[J]. Biomed Res Int, 2013, 2013: 814876. DOI: 10.1155/2013/814876. [20] ESLAM M, HASHEM AM, ROMERO-GOMEZ M, et al. FibroGENE: A gene-based model for staging liver fibrosis[J]. J Hepatol, 2016, 64(2): 390-398. DOI: 10.1016/j.jhep.2015.11.008. [21] WEI R, WANG J, WANG X, et al. Clinical prediction of HBV and HCV related hepatic fibrosis using machine learning[J]. EBioMedicine, 2018, 35: 124-132. DOI: 10.1016/j.ebiom.2018.07.041. [22] FU TT, YAO Z, DING H, et al. Computer-aided assessment of liver fibrosis progression in patients with chronic hepatitis B: An exploratory[J]. Natl Med J China, 2019, 99(7): 491-495. DOI: 10.3760/cma.j.issn.0376-2491.2019.07.003.付甜甜, 姚钊, 丁红, 等. 计算机辅助诊断慢性乙肝患者肝纤维化进程的价值分析[J]. 中华医学杂志, 2019, 99 (7): 491-495. DOI: 10.3760/cma.j.issn.0376-2491.2019.07.003. [23] CAO Y, HU ZD, LIU XF, et al. An MLP classifier for prediction of HBV-induced liver cirrhosis using routinely available clinical parameters[J]. Dis Markers, 2013, 35(6): 653-660. DOI: 10.1155/2013/127962. [24] SANG C, XIE GX, LIANG DD, et al. Improvement of liver fibrosis diagnostic models based on Youden index[J]. J Shanghai Jiaotong Univ(Med Sci), 2019, 39(10): 1156-1161. DOI: 10.3969/j.issn.1674-8115.2019.10.009.桑潮, 谢国祥, 梁丹丹, 等. 基于约登指数的肝纤维化诊断模型改进研究[J]. 上海交通大学学报(医学版), 2019, 39 (10): 1156-1161. DOI: 10.3969/j.issn.1674-8115.2019.10.009. [25] XIE G, WANG X, WEI R, et al. Serum metabolite profiles are associated with the presence of advanced liver fibrosis in Chinese patients with chronic hepatitis B viral infection[J]. BMC Med, 2020, 18(1): 144. DOI: 10.1186/s12916-020-01595-w. [26] ESTEVEZ J, CHEN VL, PODLAHA O, et al. Differential serum cytokine profiles in patients with chronic hepatitis B, C, and hepatocellular carcinoma[J]. Sci Rep, 2017, 7(1): 11867. DOI: 10.1038/s41598-017-11975-7. [27] LIAO H, XIONG T, PENG J, et al. Classification and prognosis prediction from histopathological images of hepatocellular carcinoma by a fully automated pipeline based on machine learning[J]. Ann Surg Oncol, 2020, 27(7): 2359-2369. DOI: 10.1245/s10434-019-08190-1. [28] TAO K, BIAN Z, ZHANG Q, et al. Machine learning-based genome-wide interrogation of somatic copy number aberrations in circulating tumor DNA for early detection of hepatocellular carcinoma[J]. EBioMedicine, 2020, 56: 102811. DOI: 10.1016/j.ebiom.2020.102811. [29] ZHAO RH, SHI Y, ZHAO H, et al. Acute-on-chronic liver failure in chronic hepatitis B: An update[J]. Expert Rev Gastroenterol Hepatol, 2018, 12(4): 341-350. DOI: 10.1080/17474124.2018.1426459. [30] SHI KQ, ZHOU YY, YAN HD, et al. Classification and regression tree analysis of acute-on-chronic hepatitis B liver failure: Seeing the forest for the trees[J]. J Viral Hepat, 2017, 24(2): 132-140. DOI: 10.1111/jvh.12617. [31] HERNAEZ R, SOLÀ E, MOREAU R, et al. Acute-on-chronic liver failure: An update[J]. Gut, 2017, 66(3): 541-553. DOI: 10.1136/gutjnl-2016-312670. [32] LI N, ZHENG RJ, JIE FR, et al. Analysis of the influencing factors of short-term mortality of HBV-ACLF and the establishment and comparison of prognosis models[J]. Chin Hepatol, 2019, 24 (12): 1399-1402. DOI: 10.14000/j.cnki.issn.1008-1704.2019.12.013.李楠, 郑嵘炅, 揭方荣, 等. HBV-ACLF短期死亡影响因素分析及预后模型的建立与比较研究[J]. 肝脏, 2019, 24 (12): 1399-1402. DOI: 10.14000/j.cnki.issn.1008-1704.2019.12.013. [33] CUTILLO CM, SHARMA KR, FOSCHINI L, et al. Machine intelligence in healthcare-perspectives on trustworthiness, explainability, usability, and transparency[J]. NPJ Digit Med, 2020, 3: 47. DOI: 10.1038/s41746-020-0254-2. [34] AL'AREF SJ, ANCHOUCHE K, SINGH G, et al. Clinical applications of machine learning in cardiovascular disease and its relevance to cardiac imaging[J]. Eur Heart J, 2019, 40(24): 1975-1986. DOI: 10.1093/eurheartj/ehy404. [35] LUO ZW, CHEN X, ZHANG YF, et al. Application value of machine learning algorithms and COX nomogram in the survival prediction of hepatocellular carcinoma after resection[J]. Chin J Dig Surg, 2020, 19(2): 166-178. DOI: 10.3760/cma.j.issn.1673-9752.2020.02.009.罗治文, 陈晓, 张业繁, 等. 机器学习算法和COX列线图在肝细胞癌术后生存预测中的应用价值[J]. 中华消化外科杂志, 2020, 19 (2): 166-178. DOI: 10.3760/cma.j.issn.1673-9752.2020.02.009. -
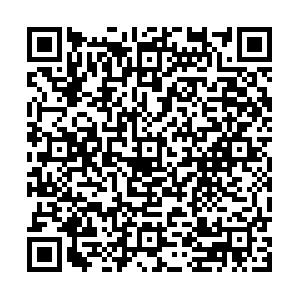
计量
- 文章访问数: 500
- HTML全文浏览量: 148
- PDF下载量: 50
- 被引次数: 0