肝胆肿瘤模型与精准药物筛选新技术
DOI: 10.3969/j.issn.1001-5256.2022.03.005
利益冲突声明: 所有作者均声明不存在利益冲突。
作者贡献声明: 刘壮负责撰写论文,文献筛选,整理数据和修改论文; 高栋负责拟定写作思路,指导撰写论文及并最终定稿。
-
摘要: 肝胆肿瘤是包括原发性肝癌、胆管癌和胆囊癌在内的恶性肿瘤。目前,肝胆肿瘤已成为世界范围内癌症相关死亡的第二大病因,而对于肝胆肿瘤的治疗手段仍无法有效应对临床需求。因此,探索开发肝胆肿瘤精准药物筛选的实验技术,寻找临床治疗新策略新方法,为早期诊断和综合治疗提供新思路,是当前领域内的关键问题。本文主要围绕肝胆肿瘤个性化病理模型的构建及药物筛选方案,特异靶点药物的设计与筛选策略,基于人工智能和大数据分析的药物筛选策略等方面介绍肝胆肿瘤精准药物筛选新技术的最新研究进展,探讨其应用潜力,并展望未来发展方向。
-
关键词:
- 肝肿瘤 /
- 胆道肿瘤 /
- 人工智能 /
- 药物筛选试验, 抗肿瘤
Abstract: Hepatobiliary tumor is a type of malignant tumor including primary liver cancer, cholangiocarcinoma, and gallbladder carcinoma. At present, hepatobiliary tumors have become the second leading cause of cancer-related death worldwide, while the treatment methods for such tumors cannot effectively meet clinical needs. Therefore, it is a key scientific problem in this field to explore and develop the experimental technology of accurate drug screening for hepatobiliary tumors, find new strategies and methods for clinical treatment, and provide new ideas for early diagnosis and comprehensive treatment of hepatobiliary tumors. This article introduces the latest research advances in the novel technologies for accurate drug screening for hepatobiliary tumor and their application potential by focusing on the construction of individualized pathological models of hepatobiliary tumor, drug screening technologies, the design and screening strategy of specific target drugs, and drug screening strategy based on artificial intelligence and big data analysis, as well as the directions for future development. -
[1] KOPETZ S, LEMOS R, POWIS G. The promise of patient-derived xenografts: The best laid plans of mice and men[J]. Clin Cancer Res, 2012, 18(19): 5160-5162. DOI: 10.1158/1078-0432.CCR-12-2408. [2] BRUNA A, RUEDA OM, GREENWOOD W, et al. A biobank of breast cancer explants with preserved intra-tumor heterogeneity to screen anticancer compounds[J]. Cell, 2016, 167(1): 260-274. e22. DOI: 10.1016/j.cell.2016.08.041. [3] CHOI SY, LIN D, GOUT PW, et al. Lessons from patient-derived xenografts for better in vitro modeling of human cancer[J]. Adv Drug Deliv Rev, 2014, 79-80: 222-237. DOI: 10.1016/j.addr.2014.09.009. [4] HAN Y, DUAN X, YANG L, et al. Identification of SARS-CoV-2 inhibitors using lung and colonic organoids[J]. Nature, 2021, 589(7841): 270-275. DOI: 10.1038/s41586-020-2901-9. [5] van de WETERING M, FRANCIES HE, FRANCIS JM, et al. Prospective derivation of a living organoid biobank of colorectal cancer patients[J]. Cell, 2015, 161(4): 933-945. DOI: 10.1016/j.cell.2015.03.053. [6] van TIENDEREN GS, GROOT KOERKAMP B, IJZERMANS J, et al. Recreating tumour complexity in a dish: Organoid models to study liver cancer cells and their extracellular environment[J]. Cancers (Basel), 2019, 11(11): 1706. DOI: 10.3390/cancers11111706. [7] BRESNAHAN E, RAMADORI P, HEIKENWALDER M, et al. Novel patient-derived preclinical models of liver cancer[J]. J Hepatol, 2020, 72(2): 239-249. DOI: 10.1016/j.jhep.2019.09.028. [8] KISSEL M, BERNDT S, FIEBIG L, et al. Antitumor effects of regorafenib and sorafenib in preclinical models of hepatocellular carcinoma[J]. Oncotarget, 2017, 8(63): 107096-107108. DOI: 10.18632/oncotarget.22334. [9] LV H, WANG C, FANG T, et al. Vitamin C preferentially kills cancer stem cells in hepatocellular carcinoma via SVCT-2[J]. NPJ Precis Oncol, 2018, 2(1): 1. DOI: 10.1038/s41698-017-0044-8. [10] MATSUKI M, HOSHI T, YAMAMOTO Y, et al. Lenvatinib inhibits angiogenesis and tumor fibroblast growth factor signaling pathways in human hepatocellular carcinoma models[J]. Cancer Med, 2018, 7(6): 2641-2653. DOI: 10.1002/cam4.1517. [11] KEATING GM, SANTORO A. Sorafenib: A review of its use in advanced hepatocellular carcinoma[J]. Drugs, 2009, 69(2): 223-240. DOI: 10.2165/00003495-200969020-00006. [12] CHEN KF, CHEN HL, TAI WT, et al. Activation of phosphatidylinositol 3-kinase/Akt signaling pathway mediates acquired resistance to sorafenib in hepatocellular carcinoma cells[J]. J Pharmacol Exp Ther, 2011, 337(1): 155-161. DOI: 10.1124/jpet.110.175786. [13] WU CX, WANG XQ, CHOK SH, et al. Blocking CDK1/PDK1/β-Catenin signaling by CDK1 inhibitor RO3306 increased the efficacy of sorafenib treatment by targeting cancer stem cells in a preclinical model of hepatocellular carcinoma[J]. Theranostics, 2018, 8(14): 3737-3750. DOI: 10.7150/thno.25487. [14] WANG F, BANK T, MALNASSY G, et al. Inhibition of insulin-like growth factor 1 receptor enhances the efficacy of sorafenib in inhibiting hepatocellular carcinoma cell growth and survival[J]. Hepatol Commun, 2018, 2(6): 732-746. DOI: 10.1002/hep4.1181. [15] LIANG Y, CHEN J, YU Q, et al. Phosphorylated ERK is a potential prognostic biomarker for Sorafenib response in hepatocellular carcinoma[J]. Cancer Med, 2017, 6(12): 2787-2795. DOI: 10.1002/cam4.1228. [16] HU B, LI H, GUO W, et al. Establishment of a hepatocellular carcinoma patient-derived xenograft platform and its application in biomarker identification[J]. Int J Cancer, 2020, 146(6): 1606-1617. DOI: 10.1002/ijc.32564. [17] JUNG J, SEOL HS, CHANG S. The Generation and application of patient-derived xenograft model for cancer research[J]. Cancer Res Treat, 2018, 50(1): 1-10. DOI: 10.4143/crt.2017.307. [18] SMITH DJ, LIN LJ, MOON H, et al. Propagating humanized BLT mice for the study of human immunology and immunotherapy[J]. Stem Cells Dev, 2016, 25(24): 1863-1873. DOI: 10.1089/scd.2016.0193. [19] WANG H, ZHOU L, XIE K, et al. Polylactide-tethered prodrugs in polymeric nanoparticles as reliable nanomedicines for the efficient eradication of patient-derived hepatocellular carcinoma[J]. Theranostics, 2018, 8(14): 3949-3963. DOI: 10.7150/thno.26161. [20] GU Q, ZHANG B, SUN H, et al. Genomic characterization of a large panel of patient-derived hepatocellular carcinoma xenograft tumor models for preclinical development[J]. Oncotarget, 2015, 6(24): 20160-20176. DOI: 10.18632/oncotarget.3969. [21] XIN H, WANG K, HU G, et al. Establishment and characterization of 7 novel hepatocellular carcinoma cell lines from patient-derived tumor xenografts[J]. PLoS One, 2014, 9(1): e85308. DOI: 10.1371/journal.pone.0085308. [22] KAPAŁCZYŹ SKA M, KOLENDA T, PRZYBYŁAW, et al. 2D and 3D cell cultures - a comparison of different types of cancer cell cultures[J]. Arch Med Sci, 2018, 14(4): 910-919. DOI: 10.5114/aoms.2016.63743. [23] DUVAL K, GROVER H, HAN LH, et al. Modeling physiological events in 2D vs. 3D cell culture[J]. Physiology (Bethesda), 2017, 32(4): 266-277. DOI: 10.1152/physiol.00036.2016. [24] IMAMURA Y, MUKOHARA T, SHIMONO Y, et al. Comparison of 2D- and 3D-culture models as drug-testing platforms in breast cancer[J]. Oncol Rep, 2015, 33(4): 1837-1843. DOI: 10.3892/or.2015.3767. [25] LANCASTER MA, KNOBLICH JA. Organogenesis in a dish: Modeling development and disease using organoid technologies[J]. Science, 2014, 345(6194): 1247125. DOI: 10.1126/science.1247125. [26] SATO T, VRIES RG, SNIPPERT HJ, et al. Single Lgr5 stem cells build crypt-villus structures in vitro without a mesenchymal niche[J]. Nature, 2009, 459(7244): 262-265. DOI: 10.1038/nature07935. [27] KARTHAUS WR, IAQUINTA PJ, DROST J, et al. Identification of multipotent luminal progenitor cells in human prostate organoid cultures[J]. Cell, 2014, 159(1): 163-175. DOI: 10.1016/j.cell.2014.08.017. [28] GAO D, VELA I, SBONER A, et al. Organoid cultures derived from patients with advanced prostate cancer[J]. Cell, 2014, 159(1): 176-187. DOI: 10.1016/j.cell.2014.08.016. [29] LOSIC B, CRAIG AJ, VILLACORTA-MARTIN C, et al. Intratumoral heterogeneity and clonal evolution in liver cancer[J]. Nat Commun, 2020, 11(1): 291. DOI: 10.1038/s41467-019-14050-z. [30] LI L, WANG H. Heterogeneity of liver cancer and personalized therapy[J]. Cancer Lett, 2016, 379(2): 191-197. DOI: 10.1016/j.canlet.2015.07.018. [31] BROUTIER L, MASTROGIOVANNI G, VERSTEGEN MM, et al. Human primary liver cancer-derived organoid cultures for disease modeling and drug screening[J]. Nat Med, 2017, 23(12): 1424-1435. DOI: 10.1038/nm.4438. [32] NUCIFORO S, FOFANA I, MATTER MS, et al. Organoid models of human liver cancers derived from tumor needle biopsies[J]. Cell Rep, 2018, 24(5): 1363-1376. DOI: 10.1016/j.celrep.2018.07.001. [33] SABOROWSKI A, WOLFF K, SPIELBERG S, et al. Murine liver organoids as a genetically flexible system to study liver cancer in vivo and in vitro[J]. Hepatol Commun, 2019, 3(3): 423-436. DOI: 10.1002/hep4.1312. [34] TAKAI A, FAKO V, DANG H, et al. Three-dimensional organotypic culture models of human hepatocellular carcinoma[J]. Sci Rep, 2016, 6: 21174. DOI: 10.1038/srep21174. [35] CAO W, LIU J, WANG L, et al. Modeling liver cancer and therapy responsiveness using organoids derived from primary mouse liver tumors[J]. Carcinogenesis, 2019, 40(1): 145-154. DOI: 10.1093/carcin/bgy129. [36] LI L, KNUTSDOTTIR H, HUI K, et al. Human primary liver cancer organoids reveal intratumor and interpatient drug response heterogeneity[J]. JCI Insight, 2019, 4(2): e121490. DOI: 10.1172/jci.insight.121490. [37] LI L, HALPERT G, LERNER MG, et al. Protein synthesis inhibitor omacetaxine is effective against hepatocellular carcinoma[J]. JCI Insight, 2021, 6(12): e138197. DOI: 10.1172/jci.insight.138197. [38] ZUCMAN-ROSSI J, VILLANUEVA A, NAULT JC, et al. Genetic landscape and biomarkers of hepatocellular carcinoma[J]. Gastroenterology, 2015, 149(5): 1226-1239. e4. DOI: 10.1053/j.gastro.2015.05.061. [39] COPUR MS. Sorafenib in advanced hepatocellular carcinoma[J]. N Engl J Med, 2008, 359(23): 2498; author reply 2498-2499. DOI: 10.1056/NEJMoa0708857 [40] ABOU-ALFA GK, JOHNSON P, KNOX JJ, et al. Doxorubicin plus sorafenib vs doxorubicin alone in patients with advanced hepatocellular carcinoma: A randomized trial[J]. JAMA, 2010, 304(19): 2154-2160. DOI: 10.1001/jama.2010.1672. [41] QIN S, BAI Y, LIM HY, et al. Randomized, multicenter, open-label study of oxaliplatin plus fluorouracil/leucovorin versus doxorubicin as palliative chemotherapy in patients with advanced hepatocellular carcinoma from Asia[J]. J Clin Oncol, 2013, 31(28): 3501-3508. DOI: 10.1200/JCO.2012.44.5643. [42] SCHULZE K, IMBEAUD S, LETOUZÉ E, et al. Exome sequencing of hepatocellular carcinomas identifies new mutational signatures and potential therapeutic targets[J]. Nat Genet, 2015, 47(5): 505-511. DOI: 10.1038/ng.3252. [43] WAGHRAY A, MURALI AR, MENON KN. Hepatocellular carcinoma: From diagnosis to treatment[J]. World J Hepatol, 2015, 7(8): 1020-1029. DOI: 10.4254/wjh.v7.i8.1020. [44] VILGRAIN V, PEREIRA H, ASSENAT E, et al. Efficacy and safety of selective internal radiotherapy with yttrium-90 resin microspheres compared with sorafenib in locally advanced and inoperable hepatocellular carcinoma (SARAH): An open-label randomised controlled phase 3 trial[J]. Lancet Oncol, 2017, 18(12): 1624-1636. DOI: 10.1016/S1470-2045(17)30683-6. [45] ARAO T, UESHIMA K, MATSUMOTO K, et al. FGF3/FGF4 amplification and multiple lung metastases in responders to sorafenib in hepatocellular carcinoma[J]. Hepatology, 2013, 57(4): 1407-1415. DOI: 10.1002/hep.25956. [46] TEYATEETI A, MAHVASH A, LONG JP, et al. Survival outcomes for yttrium-90 transarterial radioembolization with and without sorafenib for unresectable hepatocellular carcinoma patients[J]. J Hepatocell Carcinoma, 2020, 7: 117-131. DOI: 10.2147/JHC.S248314. [47] TANNIR NM, MOTZER RJ, AGARWAL N, et al. CANTATA: A randomized phase 2 study of CB-839 in combination with cabozantinib vs. placebo with cabozantinib in patients with advanced/metastatic renal cell carcinoma[J]. J Clin Oncol, 2018, 36(15): TPS4601. http://www.researchgate.net/publication/327452792_CANTATA_A_randomized_phase_2_study_of_CB-839_in_combination_with_cabozantinib_vs_placebo_with_cabozantinib_in_patients_with_advancedmetastatic_renal_cell_carcinoma [48] YAO Z, LI J, GUAN Z, et al. Liver disease screening based on densely connected deep neural networks[J]. Neural Netw, 2020, 123: 299-304. DOI: 10.1016/j.neunet.2019.11.005. [49] TAO K, BIAN Z, ZHANG Q, et al. Machine learning-based genome-wide interrogation of somatic copy number aberrations in circulating tumor DNA for early detection of hepatocellular carcinoma[J]. EBioMedicine, 2020, 56: 102811. DOI: 10.1016/j.ebiom.2020.102811. [50] SINGAL AG, MUKHERJEE A, ELMUNZER BJ, et al. Machine learning algorithms outperform conventional regression models in predicting development of hepatocellular carcinoma[J]. Am J Gastroenterol, 2013, 108(11): 1723-1730. DOI: 10.1038/ajg.2013.332. [51] JONAS S, BECHSTEIN WO, STEINMVLLER T, et al. Vascular invasion and histopathologic grading determine outcome after liver transplantation for hepatocellular carcinoma in cirrhosis[J]. Hepatology, 2001, 33(5): 1080-1086. DOI: 10.1053/jhep.2001.23561. [52] CUCCHETTI A, PISCAGLIA F, GRIGIONI AD, et al. Preoperative prediction of hepatocellular carcinoma tumour grade and micro-vascular invasion by means of artificial neural network: a pilot study[J]. J Hepatol, 2010, 52(6): 880-888. DOI: 10.1016/j.jhep.2009.12.037. [53] XU X, ZHANG HL, LIU QP, et al. Radiomic analysis of contrast-enhanced CT predicts microvascular invasion and outcome in hepatocellular carcinoma[J]. J Hepatol, 2019, 70(6): 1133-1144. DOI: 10.1016/j.jhep.2019.02.023. [54] DONG Y, ZHOU L, XIA W, et al. Preoperative prediction of microvascular invasion in hepatocellular carcinoma: Initial application of a radiomic algorithm based on grayscale ultrasound images[J]. Front Oncol, 2020, 10: 353. DOI: 10.3389/fonc.2020.00353. [55] ZHOU W, ZHANG L, WANG K, et al. Malignancy characterization of hepatocellular carcinomas based on texture analysis of contrast-enhanced MR images[J]. J Magn Reson Imaging, 2017, 45(5): 1476-1484. DOI: 10.1002/jmri.25454. -
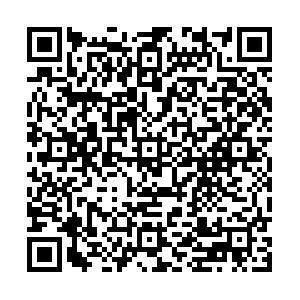
表(1)
计量
- 文章访问数: 824
- HTML全文浏览量: 149
- PDF下载量: 133
- 被引次数: 0