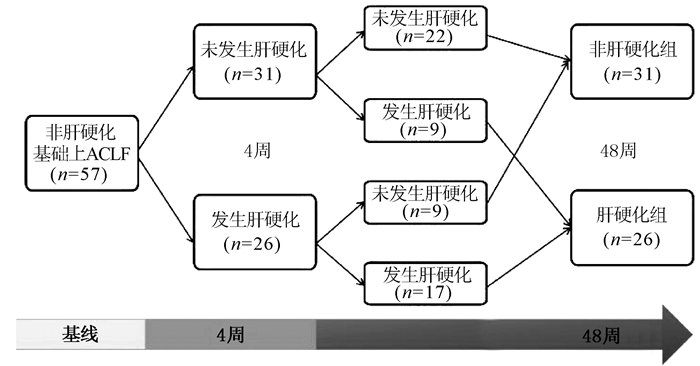
A large amount of information,such as clinical hematological data and imaging images,can be extracted by artificial intelligence to form various quantifiable features,analyze the association between different features and problems concerned( such as diagnosis),and thus solve complex medical problems. This article elaborates on the efficiency of various artificial intelligence algorithms in the diagnosis of pancreatic cancer,hepatic fibrosis,and esophageal varices,so as to help clinicians with clearer understanding and better decision-making.
慢加急性肝衰竭(ACLF)是在慢性肝炎或肝硬化基础上出现急性肝功能失代偿。临床工作中发现,随着急性肝损伤的减轻和时间的推移,部分非肝硬化基础上ACLF患者肝功能逐渐恢复,但部分患者会逐渐出现非可逆性肝硬化。以往学者更多关注ACLF的短期存活率[1-3],临床实际中,存活的ACLF恢复期患者预后同样值得关注,本研究通过动态观察非肝硬化基础上ACLF恢复期患者的临床及影像学检查结果,初步探索了影响非肝硬化基础上ACLF恢复期患者在48周发生肝硬化的危险因素,有望为指导ACLF恢复期患者的随访管理提供依据。
选取2015年1月—2019年6月于首都医科大学附属北京佑安医院疑难肝病及人工肝科收治的ACLF患者,以首次诊断ACLF的时间为基线,所有患者入院后均接受标准化治疗,通过查阅电子病历及电话随访获取患者的预后情况。纳入标准:(1)符合亚太肝病学会(APASL)发布的ACLF诊断标准[2],在慢性肝病基础上出现急性肝损伤症候群,表现为4周内出现黄疸(TBil≥85 μmol/L),凝血异常(INR≥1.5),伴随腹水和/或肝性脑病;(2)随访时间大于48周且临床资料齐全。排除标准:(1)肝硬化基础上的ACLF患者;(2)随访期间行肝移植术者;(3)临床病例资料缺失者;(4)拒绝随访或电话信息缺失者。根据纳入和排除标准,最终纳入了57例非肝硬化基础上ACLF患者。根据48周有无发生肝硬化分为非肝硬化组和肝硬化组。
ACLF恢复参考APASL-ACLF专家共识[2]:乏力及消化道症状基本消失,TBil下降至≤85 μmol/L,INR<1.5,无肝性脑病及明显腹水。
收集患者的性别、年龄、肝病基础、并发症、入院时各项相关临床指标、影像学检查(CT或MRI)结果等,并记录患者随访期间的临床相关数据。根据提取的临床数据和资料,计算患者基线MELD和MELD-Na评分以及无创肝纤维化评分Sheth、AST和PLT比值指数(APRI)和肝纤维化4因子(FIB-4)指数。Sheth指数=AST /ALT;APRI指数=(AST/正常值上限×100)/PLT;FIB-4指数=(年龄×AST)/(PLT ×ALT1/2)。通过临床及影像学检查判断基线、距离基线4周和48周时患者是否发生肝硬化情况。肝硬化影像学显示肝脏形态不规则,边缘呈波浪状,肝叶比例失调,部分肝节段明显萎缩,肝裂显著增宽且不规则[4]。
本研究方案经由首都医科大学附属北京佑安医院伦理委员会审批,批号:LL-2020-178-K。
采用R软件(版本号R×64 4.0.3)对数据进行统计分析,正态分布计量资料以x ±s表示,两组间比较采用t检验,非正态分布计量资料以M(P25~P75)表示,两组间比较采用Mann-Whitney U检验;计数资料两组间比较采用χ2检验或Fisher精确检验。将组间比较P<0.05的因素纳入logistic单因素及多因素分析,并通过经受试者工作特征曲线(ROC曲线)评估独立危险因素的预测效能。P<0.05为差异有统计学意义。
57例非肝硬化基础上ACLF患者中男性居多,为49例(85.9%);慢性肝病病因有慢性乙型肝炎44例、酒精性肝病6例、慢性乙型肝炎合并酒精性肝病3例、药物性肝病2例、慢性乙型肝炎合并非酒精性脂肪肝1例,不明原因肝病1例。
在57例恢复期患者中,经动态观察发现,有26例患者在随访4周内有肝硬化表现,其中9例(34.6%)患者在随访48周时发现肝硬化消失;17例患者持续肝硬化状态,具体的动态过程详见图 1,最终在48周进展至肝硬化的患者有26例(45.6%)。
肝硬化组中ChE和PLT均显著低于非肝硬化组,差异均具有统计学意义(P值均<0.05);两项肝衰竭预后评分及三项无创肝纤维化评分比较,肝硬化组FIB-4指数显著高于非肝硬化组,差异具有统计学意义(P<0.05)。年龄、性别、肝衰竭并发症方面在两组患者中的分布差异均无统计学意义,两组患者的ALT、AST、ALP、GGT、Alb、TBil、INR、Cr、Na、AFP、Hb、WBC水平差异均无统计学意义(P值均>0.05)(表 1)。
项目 | 非肝硬化组(n=31) | 肝硬化组(n=26) | 统计值 | P值 |
男[例(%)] | 27(87.1) | 22(84.6) | 1.000 | |
年龄(岁) | 39.61±10.65 | 43.50±10.60 | t=-1.376 | 0.175 |
并发症[例(%)] | ||||
自发性腹膜炎 | 29(93.5) | 23(88.5) | 0.651 | |
腹水 | 17(54.8) | 19(73.1) | χ2=1.314 | 0.252 |
肝性脑病 | 5(16.1) | 1(3.8) | 0.205 | |
急性肾损伤 | 3(9.7) | 2(7.7) | 1.000 | |
上消化道出血 | 1(3.2) | 0 | 1.000 | |
ALT(U/L) | 388.05(140.70~1330.53) | 189.60(81.72~507.97) | Z=497.0 | 0.080 |
AST(U/L) | 250.45(118.35~592.58) | 197.40(98.92~406.53) | Z=450.5 | 0.324 |
ALP(U/L) | 141.00(106.80~164.20) | 138.30(120.00~160.70) | Z=197.5 | 0.968 |
GGT(U/L) | 123.90(80.10~152.50) | 79.30(56.00~146.70) | Z=306.0 | 0.207 |
Alb(g/L) | 31.71±4.73 | 30.58±4.38 | t=0.921 | 0.361 |
TBil(μmol/L) | 288.74±137.39 | 319.45±132.47 | t=-0.857 | 0.395 |
ChE(U/L) | 4137.59±1604.83 | 2844.32±961.05 | t=3.177 | 0.003 |
INR | 2.21 (1.90~2.84) | 2.12 (1.73~2.45) | Z=474.0 | 0.259 |
Cr(μmol/L) | 56.10(47.80~66.50) | 63.50(52.25~71.75) | Z=344.5 | 0.353 |
Na(mmol/L) | 136.95±4.17 | 136.15±3.27 | t=0.818 | 0.417 |
AFP(ng/ml) | 86.42(32.79~185.45) | 88.67(17.68~177.42) | Z=353.0 | 0.435 |
Hb(g/L) | 133.00±19.50 | 123.50±20.85 | t=1.752 | 0.086 |
WBC(×109/L) | 7.20(5.35~8.86) | 6.44(4.90~9.30) | Z=446.0 | 0.496 |
PLT(×109/L) | 138.84±56.46 | 100.04±57.28 | t=2.564 | 0.013 |
肝纤维化评分 | ||||
Sheth指数 | 0.65(0.49~1.05) | 1.07(0.55~1.55) | Z=295.0 | 0.121 |
APRI指数 | 6.35(2.25~10.42) | 4.93(2.96~9.04) | Z=365.0 | 0.690 |
FIB-4指数 | 4.45(2.14~7.80) | 7.81(3.92~11.36) | Z=258.0 | 0.030 |
肝衰竭预后评分 | ||||
MELD-Na评分 | 21.33±3.75 | 20.49±4.42 | t=0.754 | 0.455 |
MELD评分 | 18.04(13.48~22.00) | 16.67(14.16~22.76) | Z=370.0 | 0.781 |
将组间比较结果中具有统计学差异的指标纳入logistic回归分析,结果显示低水平的ChE和PLT是非肝硬化基础上ACLF恢复期患者发生肝硬化的独立危险因素(P值均<0.05)(表 2)。
变量 | 单因素分析 | 多因素分析 | |||
OR(95%CI) | P值 | OR(95%CI) | P值 | ||
ChE | 1.001(1.000~1.002) | 0.011 | 1.001(1.000~1.002) | 0.010 | |
PLT | 1.013(1.002~1.024) | 0.020 | 1.015(1.002~1.028) | 0.027 | |
FIB-4指数 | 0.933(0.847~1.027) | 0.156 |
经ROC曲线分析结果显示,ChE预测非肝硬化基础上ACLF患者48周发生肝硬化的曲线下面积(AUC)为0.761(95%CI: 0.613~0.908) (P=0.004);PLT预测的AUC为0.702(95%CI: 0.538~0.866)(P=0.027);两项指标联合预测的AUC为0.835(95%CI: 0.713~0.957)(P<0.001),提示两项指标联合在肝硬化的预测方面具有更大的效能(图 2)。
ACLF疾病发生发展过程中存在肝细胞大量坏死,在疾病恢复过程中,会发现部分原本无肝硬化基础的患者会逐渐出现肝硬化情况,其发生率尚不明确,缺乏简单而准确的指标或评分来提前识别这些患者,也缺乏规范实用的ACLF恢复期管理方案。
本研究发现57例非肝硬化基础上ACLF患者中随访至48周有26例(45.6%)患者发生肝炎坏死后肝硬化,这与国内一项研究[5]结果相似,研究者对65例CHB基础上ACLF随访3个月,发现有30例(46%)患者发生肝炎坏死后肝硬化。这提示有近50%的非肝硬化基础上ACLF患者在1年内进展为肝硬化,关注这一类患者的预警危险因素对临床指导至关重要。本研究发现,57例患者中有9例在随访4周内发生肝硬化,但随访48周肝硬化消失,提示在肝衰竭疾病早期,部分患者因严重肝损伤,其影像学可能表现为肝硬化征象,而通过恢复期的肝脏修复,这种肝硬化是可逆的。有学者[6]通过分析乙型肝炎相关ACLF患者的CT表现发现,47.4%的ACLF患者肝脏体积缩小,以中晚期患者为主,病程超过数周肝内可形成结节样再生,因此肝衰竭患者在病程不同时期表现为不同的CT特征性表现。在患者恢复的过程中如残留肝细胞增生、肝脏再生能力较强的情况下,肝脏生化指标恢复的同时,肝脏影像学也会改善[7]。另外,有9例在随访4周内没有出现肝硬化,但随访48周发生肝硬化。部分患者虽然在疾病早期没有表现为肝硬化,但因肝损伤严重,在修复过程中逐渐出现了肝硬化。由于患者入院时往往处于ACLF病程不同阶段,因此表现出不同影像学特征,故在肝衰竭疾病早期评估有无肝硬化尚不准确,据此笔者将评估终点定在距离基线48周。通过对多项指标的单因素及多因素分析,发现ChE和PLT是非肝硬化基础上ACLF恢复期患者48周时发生肝硬化的独立危险因素,两者联合预测更有优势。
肝脏是合成血清ChE的唯一场所,ChE受肝脏合成和分解代谢的影响。在急性病毒性肝炎、慢性活动性肝炎、失代偿期肝硬化以及肝衰竭等肝脏疾病中均可检测到不同程度ChE的降低。因此血清ChE是一个评估肝脏储备功能极为敏感、稳定的指标,不仅反映肝脏的合成功能,且反映肝组织损伤的程度,是判断慢性肝病转归的良好指标[8]。ChE与MELD评分联合应用,可减少肝外因素造成的MELD评分误差,提高其对肝脏储备功能评价的精准度,对肝病患者的预后判断具有重要价值[9]。GGT-PLT比值(GPR)可用于预测慢性乙型肝炎(CHB)的明显纤维化和肝硬化方面,近期国内一项大型队列研究[10]证实,GPR指数在增加ChE参数后,具有更高的评估性能。此外,有研究者[11]开发了融合游离四碘甲状腺素、PLT、ChE、GGT和年龄等参数的FibroStage评分方法,证实与APRI、FIB-4、Lok等指数比较,FibroStage可更好地用于全面诊断显著肝纤维化、晚期肝纤维化和肝硬化。由于ChE不易受黄疸、输血制品等其他因素影响,稳定性好,未来可作为预测肝脏疾病进展和预后的重要指标之一。
PLT具有在内皮破裂或血管损伤时介导止血的作用外,还是与肝损伤程度、纤维化阶段及疾病预后密切相关的参数[12]。肝修复和再生需要促增殖和抗增殖信号来协调组织修复,研究[13-14]显示PLT在对肝细胞产生促有丝分裂作用的同时,可抑制纤维化形成并启动纤维溶解途径。诱导慢性胆汁淤积小鼠PLT减少会导致肝纤维化,从而使肝功能恶化[15-16]。人类PLT可通过分泌肝细胞生长因子抑制严重联合免疫缺陷小鼠的肝纤维化,HGF通过同时阻止肝星状细胞激活和促进基质金属蛋白酶9的表达来发挥抗纤维化作用[17-18]。
PLT是众多肝脏疾病评分中的重要参数之一,如肝纤维化评分:APRI[19]、FIB-4[20]、GPR[21]、FibroStage[11]、红细胞体积分布宽度-血小板比值[22]、球蛋白-血小板模型[23]、肝脏硬度测定值(LSM)与PLT比值[24],以及以PLT和LSM为基础的Baveno Ⅵ标准(B6C)[25]等,充分提示PLT在肝脏疾病评估和预测中的重要性,其地位不容小觑。
综上,目前越来越多研究证实ChE和PLT参数在预测肝纤维化、肝损伤程度和疾病预后方面具有重要地位。本研究也表明ChE和PLT在预测非肝硬化基础上ACLF恢复期患者48周时发生肝硬化具有一定效能,是两项独立危险因素。这两项指标检测费用低廉、且易于应用,作为非肝硬化基础ACLF恢复期患者发生肝硬化的预测指标,对此类患者个性化治疗及复诊建议有较好的指导价值。甚至对以后ACLF恢复期患者规范的随访管理流程制订有一定参考价值。
因相关临床资料完整的病例较为稀缺,本研究具有一定的局限性,是小样本、单中心、包含不同病因非肝硬化基础ACLF患者的回顾性研究,可能存在混杂因素影响统计分析结果,若想获得能够更为准确的预测指标或模型,尚需要大样本、多中心、针对不同病因的前瞻性研究加以验证及进一步探索。
[1] LE BERRE C,SANDBORN WJ,ARIDHI S,et al. Application of Artificial Intelligence to Gastroenterology and Hepatology[J]. Gastroenterology,2020,158(1):76-94. e2.
|
[2] LECUN Y,BENGIO Y,HINTON G. Deep learning[J]. Nature,2015,521(7553):436-444.
|
[3] FRITSCHER-RAVENS A,BRAND L,KNOFEL WT,et al. Comparison of endoscopic ultrasound-guided fine needle aspiration for focal pancreatic lesions in patients with normal parenchyma and chronic pancreatitis[J]. Am J Gastroenterol,2002,97(11):2768-2775.
|
[4] CASTELLANO G,BONILHA L,LI LM,et al. Texture analysis of medical images[J]. Clin Radiol,2004,59(12):1061-1069.
|
[5] DAS A,NGUYEN CC,LI F,et al. Digital image analysis of EUS images accurately differentiates pancreatic cancer from chronic pancreatitis and normal tissue[J]. Gastrointest Endosc,2008,67(6):861-867.
|
[6] ZHU M,XU C,YU J,et al. Differentiation of pancreatic cancer and chronic pancreatitis using computer-aided diagnosis of endoscopic ultrasound(EUS)images:A diagnostic test[J]. PLo S One,2013,8(5):e63820.
|
[7] OZKAN M,CAKIROGLU M,KOCAMAN O,et al. Age-based computer-aided diagnosis approach for pancreatic cancer on endoscopic ultrasound images[J]. Endosc Ultrasound,2016,5(2):101-107.
|
[8] SFTOIU A,VILMANN P,GORUNESCU F,et al. Efficacy of an artificial neural network-based approach to endoscopic ultrasound elastography in diagnosis of focal pancreatic masses[J]. Clin Gastroenterol Hepatol,2012,10(1):84-90. e1.
|
[9] SFTOIU A,VILMANN P,DIETRICH CF,et al. Quantitative contrast-enhanced harmonic EUS in differential diagnosis of focal pancreatic masses(with videos)[J]. Gastrointest Endosc,2015,82(1):59-69.
|
[10] YANG Y,CHEN H,WANG D,et al. Diagnosis of pancreatic carcinoma based on combined measurement of multiple serum tumor markers using artificial neural network analysis[J]. Chin Med J(Engl),2014,127(10):1891-1896.
|
[11] LIU SL,LI S,GUO YT,et al. Establishment and application of an artificial intelligence diagnosis system for pancreatic cancer with a faster region-based convolutional neural network[J].Chin Med J(Engl),2019,132(23):2795-2803.
|
[12] LI S,JIANG H,WANG Z,et al. An effective computer aided diagnosis model for pancreas cancer on PET/CT images[J].Comput Methods Programs Biomed,2018,165:205-214.
|
[13] GAO X,WANG X. Performance of deep learning for differentiating pancreatic diseases on contrast-enhanced magnetic resonance imaging:A preliminary study[J]. Diagn Interv Imaging,2020,101(2):91-100.
|
[14] DIEHL AM,DAY C. Cause,pathogenesis,and treatment of nonalcoholic steatohepatitis[J]. N Engl J Med,2017,377(21):2063-2072.
|
[15] PISCAGLIA F,CUCCHETTI A,BENLLOCH S,et al. Prediction of significant fibrosis in hepatitis C virus infected liver transplant recipients by artificial neural network analysis of clinical factors[J]. Eur J Gastroenterol Hepatol,2006,18(12):1255-1261.
|
[16] HASHEM S,ESMAT G,ELAKEL W,et al. Comparison of machine learning approaches for prediction of advanced liver fibrosis in chronic hepatitis C patients[J]. IEEE/ACM Trans Comput Biol Bioinform,2018,15(3):861-868.
|
[17] WANG D,WANG Q,SHAN F,et al. Identification of the risk for liver fibrosis on CHB patients using an artificial neural network based on routine and serum markers[J]. BMC Infect Dis,2010,10:251.
|
[18] WEI R,WANG J,WANG X,et al. Clinical prediction of HBV and HCV related hepatic fibrosis using machine learning[J].EBio Medicine,2018,35:124-132.
|
[19] RAOUFY MR,VAHDANI P,ALAVIAN SM,et al. A novel method for diagnosing cirrhosis in patients with chronic hepatitis B:Artificial neural network approach[J]. J Med Syst,2011,35(1):121-126.
|
[20] CHEN Y,LUO Y,HUANG W,et al. Machine-learning-based classification of real-time tissue elastography for hepatic fibrosis in patients with chronic hepatitis B[J]. Comput Biol Med,2017,89:18-23.
|
[21] WANG K,LU X,ZHOU H,et al. Deep learning Radiomics of shear wave elastography significantly improved diagnostic performance for assessing liver fibrosis in chronic hepatitis B:A prospective multicentre study[J]. Gut,2019,68(4):729-741.
|
[22] YASAKA K,AKAI H,KUNIMATSU A,et al. Liver Fibrosis:Deep convolutional neural network for staging by using gadoxetic acid-enhanced hepatobiliary phase MR images[J]. Radiology,2018,287(1):146-155.
|
[23] YASAKA K,AKAI H,KUNIMATSU A,et al. Deep learning for staging liver fibrosis on CT:A pilot study[J]. Eur Radiol,2018,28(11):4578-4585.
|
[24] SOWA JP,ATMACAKAHRAMAN A,et al. Non-invasive separation of alcoholic and non-alcoholic liver disease with predictive modeling[J]. PLo S One,2014,9(7):e101444.
|
[25] ESLAM M,NEWSOME PN,SARIN SK,et al. A new definition for metabolic dysfunction-associated fatty liver disease:An international expert consensus statement[J]. J Hepatol,2020,73(1):202-209.
|
[26] ESLAM M,SANYAL AJ,GEORGE J,et al. MAFLD:A consensus-driven proposed nomenclature for metabolic associated fatty liver disease[J]. Gastroenterology,2020,158(7):1999-2014. e1.
|
[27] GAO X. New thoughts about renaming nonalcoholic fatty liver disease[J]. J Clin Hepatol,2020,36(6):1201-1204.(in Chinese)高鑫.非酒精性脂肪性肝病更名带来的新思考[J].临床肝胆病杂志,2020,36(6):1201-1204.
|
[28] SOWA JP,HEIDER D,BECHMANN LP,et al. Novel algorithm for non-invasive assessment of fibrosis in NAFLD[J]. PLo S One,2013,8(4):e62439.
|
[29] YIP TC,MA AJ,WONG VW,et al. Laboratory parameterbased machine learning model for excluding non-alcoholic fatty liver disease(NAFLD)in the general population[J]. Aliment Pharmacol Ther,2017,46(4):447-456.
|
[30] FIALOKE S,MALARSTIG A,MILLER MR,et al. Application of machine learning methods to predict non-alcoholic steatohepatitis(NASH)in non-alcoholic fatty liver(NAFL)patients[J]. AMIA Annu Symp Proc,2018,2018:430-439.
|
[31] HAN D,QI XS,YU Y. An excerpt of portal hypertensive bleeding in cirrhosis:Risk stratification,diagnosis and management-2016practice guidance by the American Association for the Study of Liver Diseases[J]. J Clin Hepatol,2017,33(3):422-427.(in Chinese)韩丹,祁兴顺,于洋.《2016年美国肝病学会肝硬化门静脉高压出血的风险分层、诊断和管理实践指导》摘译[J].临床肝胆病杂志,2017,33(3):422-427.
|
[32] HONG WD,JI YF,WANG D,et al. Use of artificial neural network to predict esophageal varices in patients with HBV related cirrhosis[J]. Hepat Mon,2011,11(7):544-547.
|
[33] DONG TS,KALANI A,ABY ES,et al. Machine learningbased development and validation of a scoring system for screening high-risk esophageal varices[J]. Clin Gastroenterol Hepatol,2019,17(9):1894-1901. e1.
|
项目 | 非肝硬化组(n=31) | 肝硬化组(n=26) | 统计值 | P值 |
男[例(%)] | 27(87.1) | 22(84.6) | 1.000 | |
年龄(岁) | 39.61±10.65 | 43.50±10.60 | t=-1.376 | 0.175 |
并发症[例(%)] | ||||
自发性腹膜炎 | 29(93.5) | 23(88.5) | 0.651 | |
腹水 | 17(54.8) | 19(73.1) | χ2=1.314 | 0.252 |
肝性脑病 | 5(16.1) | 1(3.8) | 0.205 | |
急性肾损伤 | 3(9.7) | 2(7.7) | 1.000 | |
上消化道出血 | 1(3.2) | 0 | 1.000 | |
ALT(U/L) | 388.05(140.70~1330.53) | 189.60(81.72~507.97) | Z=497.0 | 0.080 |
AST(U/L) | 250.45(118.35~592.58) | 197.40(98.92~406.53) | Z=450.5 | 0.324 |
ALP(U/L) | 141.00(106.80~164.20) | 138.30(120.00~160.70) | Z=197.5 | 0.968 |
GGT(U/L) | 123.90(80.10~152.50) | 79.30(56.00~146.70) | Z=306.0 | 0.207 |
Alb(g/L) | 31.71±4.73 | 30.58±4.38 | t=0.921 | 0.361 |
TBil(μmol/L) | 288.74±137.39 | 319.45±132.47 | t=-0.857 | 0.395 |
ChE(U/L) | 4137.59±1604.83 | 2844.32±961.05 | t=3.177 | 0.003 |
INR | 2.21 (1.90~2.84) | 2.12 (1.73~2.45) | Z=474.0 | 0.259 |
Cr(μmol/L) | 56.10(47.80~66.50) | 63.50(52.25~71.75) | Z=344.5 | 0.353 |
Na(mmol/L) | 136.95±4.17 | 136.15±3.27 | t=0.818 | 0.417 |
AFP(ng/ml) | 86.42(32.79~185.45) | 88.67(17.68~177.42) | Z=353.0 | 0.435 |
Hb(g/L) | 133.00±19.50 | 123.50±20.85 | t=1.752 | 0.086 |
WBC(×109/L) | 7.20(5.35~8.86) | 6.44(4.90~9.30) | Z=446.0 | 0.496 |
PLT(×109/L) | 138.84±56.46 | 100.04±57.28 | t=2.564 | 0.013 |
肝纤维化评分 | ||||
Sheth指数 | 0.65(0.49~1.05) | 1.07(0.55~1.55) | Z=295.0 | 0.121 |
APRI指数 | 6.35(2.25~10.42) | 4.93(2.96~9.04) | Z=365.0 | 0.690 |
FIB-4指数 | 4.45(2.14~7.80) | 7.81(3.92~11.36) | Z=258.0 | 0.030 |
肝衰竭预后评分 | ||||
MELD-Na评分 | 21.33±3.75 | 20.49±4.42 | t=0.754 | 0.455 |
MELD评分 | 18.04(13.48~22.00) | 16.67(14.16~22.76) | Z=370.0 | 0.781 |
变量 | 单因素分析 | 多因素分析 | |||
OR(95%CI) | P值 | OR(95%CI) | P值 | ||
ChE | 1.001(1.000~1.002) | 0.011 | 1.001(1.000~1.002) | 0.010 | |
PLT | 1.013(1.002~1.024) | 0.020 | 1.015(1.002~1.028) | 0.027 | |
FIB-4指数 | 0.933(0.847~1.027) | 0.156 |
项目 | 非肝硬化组(n=31) | 肝硬化组(n=26) | 统计值 | P值 |
男[例(%)] | 27(87.1) | 22(84.6) | 1.000 | |
年龄(岁) | 39.61±10.65 | 43.50±10.60 | t=-1.376 | 0.175 |
并发症[例(%)] | ||||
自发性腹膜炎 | 29(93.5) | 23(88.5) | 0.651 | |
腹水 | 17(54.8) | 19(73.1) | χ2=1.314 | 0.252 |
肝性脑病 | 5(16.1) | 1(3.8) | 0.205 | |
急性肾损伤 | 3(9.7) | 2(7.7) | 1.000 | |
上消化道出血 | 1(3.2) | 0 | 1.000 | |
ALT(U/L) | 388.05(140.70~1330.53) | 189.60(81.72~507.97) | Z=497.0 | 0.080 |
AST(U/L) | 250.45(118.35~592.58) | 197.40(98.92~406.53) | Z=450.5 | 0.324 |
ALP(U/L) | 141.00(106.80~164.20) | 138.30(120.00~160.70) | Z=197.5 | 0.968 |
GGT(U/L) | 123.90(80.10~152.50) | 79.30(56.00~146.70) | Z=306.0 | 0.207 |
Alb(g/L) | 31.71±4.73 | 30.58±4.38 | t=0.921 | 0.361 |
TBil(μmol/L) | 288.74±137.39 | 319.45±132.47 | t=-0.857 | 0.395 |
ChE(U/L) | 4137.59±1604.83 | 2844.32±961.05 | t=3.177 | 0.003 |
INR | 2.21 (1.90~2.84) | 2.12 (1.73~2.45) | Z=474.0 | 0.259 |
Cr(μmol/L) | 56.10(47.80~66.50) | 63.50(52.25~71.75) | Z=344.5 | 0.353 |
Na(mmol/L) | 136.95±4.17 | 136.15±3.27 | t=0.818 | 0.417 |
AFP(ng/ml) | 86.42(32.79~185.45) | 88.67(17.68~177.42) | Z=353.0 | 0.435 |
Hb(g/L) | 133.00±19.50 | 123.50±20.85 | t=1.752 | 0.086 |
WBC(×109/L) | 7.20(5.35~8.86) | 6.44(4.90~9.30) | Z=446.0 | 0.496 |
PLT(×109/L) | 138.84±56.46 | 100.04±57.28 | t=2.564 | 0.013 |
肝纤维化评分 | ||||
Sheth指数 | 0.65(0.49~1.05) | 1.07(0.55~1.55) | Z=295.0 | 0.121 |
APRI指数 | 6.35(2.25~10.42) | 4.93(2.96~9.04) | Z=365.0 | 0.690 |
FIB-4指数 | 4.45(2.14~7.80) | 7.81(3.92~11.36) | Z=258.0 | 0.030 |
肝衰竭预后评分 | ||||
MELD-Na评分 | 21.33±3.75 | 20.49±4.42 | t=0.754 | 0.455 |
MELD评分 | 18.04(13.48~22.00) | 16.67(14.16~22.76) | Z=370.0 | 0.781 |
变量 | 单因素分析 | 多因素分析 | |||
OR(95%CI) | P值 | OR(95%CI) | P值 | ||
ChE | 1.001(1.000~1.002) | 0.011 | 1.001(1.000~1.002) | 0.010 | |
PLT | 1.013(1.002~1.024) | 0.020 | 1.015(1.002~1.028) | 0.027 | |
FIB-4指数 | 0.933(0.847~1.027) | 0.156 |